The forthcoming upgrade of ECMWF’s Integrated Forecasting System (IFS) to Cycle 49r1 includes observation errors diagnosed using a simpler method than previously for the Cross-track Infrared Sounder (CrIS), which is on board several American satellites. Also, a more physically consistent method of prescribing the observation error covariances for CrIS, to describe correlations between errors in the different channels, has been implemented. This is achieved by prescribing the observation errors in units of radiance, and then dynamically mapping these errors into brightness temperature units for every scene.
Diagnosing observation error covariances
For some time at ECMWF, observation error covariance matrices have been derived using the difference between observations and both a short-range forecast (the ‘background’) and the best estimate (the ‘analysis’) of the current state of the Earth system, using the method proposed by Desroziers et al., 2005 (https://doi.org/10.1256/qj.05.108). As with any method of diagnosing observation errors, some assumptions are required. These include unbiased errors in observations, short-range forecasts, and analyses. They also include the assumption that observation and background errors are uncorrelated. The Desroziers diagnostic will result in suboptimal estimation if these criteria are not met.
In practice, these conditions are not met. For a new observation type, a time-consuming set of assimilation experiments with sub-optimal error covariances is used to generate the set of background and analysis departures from observations used in the method. Even then, the resulting matrix is not symmetrical, can be badly conditioned, and is likely to need arbitrary scaling to give good forecast impact.
Our proposal is to simply use the covariance of analysis departures, which can come from an experiment with all observations actively assimilated, except, in this case, CrIS observations. The CrIS observations will be passive (i.e. monitored only and not affecting the analysis). This is effectively assuming the analysis to be a proxy for the truth. Benefits of this method include the fact that the resulting matrix is symmetrical and well-conditioned (i.e. it is a covariance matrix by definition), and that it can be applied without running an initial assimilation experiment to generate the analysis departures. No method for estimating observation errors is free from assumptions, but this method has been shown to be effective in terms of forecast impact, and it has the advantage that it can be applied quickly to observations from new instruments.
Specifying scene-dependent observation error covariances
Often, we refer to the assimilation of radiance observations, when in fact it is quantities of brightness temperature that are assimilated in the ECMWF system, having been converted using the Planck function (see the box). An attractive aspect of working in brightness temperature (units of Kelvin) is that the values are physically intuitive and closely related to geophysical temperature. In contrast, radiances have non-intuitive values, varying over several orders of magnitude depending on channel, making them an unattractive option for assimilation. Assimilating these quantities directly would involve a great deal of technical effort.
The Planck function gives the radiance spectrum emitted by an ideal black body at a particular temperature.
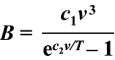
Where B is the radiance in Wm-2sr -1cm, ν is the wavenumber in cm-1, T is the temperature in Kelvin, and c1 = 2hc2 and c2 = hc/kB (h, c and kB are the Planck constant, the speed of light and the Boltzmann constant, respectively).
The derivative of this equation with respect to temperature is:
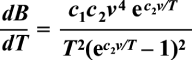
So, to convert small uncertainties in radiance units to those in units of Kelvin, for a given observed brightness temperature, we can simply calculate:
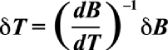
When a radiance measurement is made by a satellite, the noise characteristics are approximately Gaussian in radiance units. However, when these measurements are fed into the non‑linear Planck function, the resulting noise distribution in brightness temperature units becomes very non-Gaussian. This is particularly true at the high-frequency end of the infrared spectrum, and the non-Gaussian distribution of errors compromises one of the key assumptions in variational data assimilation.
Our suggested solution is to diagnose the observation error covariance in units of radiance, and then, at the time of the assimilation, dynamically convert these to brightness temperature units. This can be done using the derivative of the Planck function, in a way that is consistent with each scene. This compromise preserves inter-channel error correlations and allows a more appropriate specification of observation errors, while retaining the intuitive units of Kelvin for the monitoring of the observations.
Error covariances diagnosed by the new method radiance units, and dynamically mapped to brightness temperature units at the time of assimilation, have been tested for CrIS. The results are positive in the short range in terms of predictions of 500 hPa geopotential height (see the figure). This will become operational in Cycle 49r1, and it is intended that the method will be extended to other instruments in Cycle 50r1.
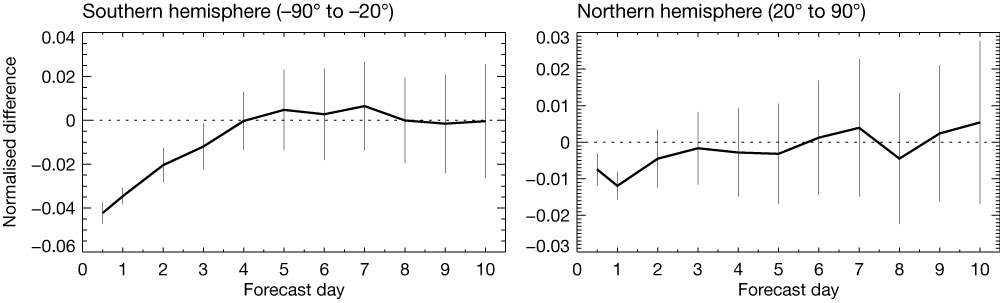