ECMWF has a long history of development and production of ocean and sea-ice reanalysis. Initially conceived to provide initial conditions for seasonal re-forecasts, the user base of the ECMWF ocean and sea-ice reanalysis has increased over the years. This reanalysis supports climate monitoring, the initialisation of extended-range and decadal forecasts, and more recently the initialisation of medium-range forecasts. The 5th generation of ECMWF’s ocean and sea-ice reanalysis system, ORAS5, went operational in 2016. It serves as the basis of OCEAN5, which has been providing ocean and sea-ice initial conditions for all ECMWF coupled forecasting systems ever since (Zuo et al., 2019). ORAS6 – the 6th generation of ocean and sea-ice ensemble reanalysis – will have an important addition: the provision of surface boundary conditions for the upcoming atmospheric reanalysis ERA6.
As a consequence, the faithful representation of sea-surface temperature (SST) and sea-ice mean state and variability, including fast processes such as the diurnal cycle, has been a priority in the development and design of ORAS6. For this to happen, variational assimilation of SST in the ocean model was implemented for the first time in the ECMWF system. This required the representation of flow-dependent error covariances via an Ensemble of Data Assimilations (EDA) in the ocean. The SST assimilation, together with the availability of ERA5 hourly surface forcing, has made it possible for ORAS6 to represent the diurnal cycle in SST, which is one of the main highlights of this new system. ORAS6 brings another important novelty: the availability of its output in the ECMWF Meteorological Archival and Retrieval System (MARS) in the generally used format for weather data, binary GRIB2 (see Sármány et al., 2024). Other innovations include: an update of the ocean and sea-ice model, the latter bringing significant physical upgrades; enhanced ocean and sea-ice observation usage; an updated bias correction scheme; and ensemble generation. All of these have been made possible by more than eight additional years of research and development at ECMWF and close collaborations with meteorological services and research institutions in ECMWF Member States. ORAS6 has been developed as a dedicated cycle of ECMWF’s Integrated Forecasting System (IFS), Cycle 48r2. The evolution of ORAS6 is strategically determined by the impact on coupled forecasting systems and performance assessments, including feedback from end user groups. A complete ORAS6 record, back to 1950, is expected to be available by the end of 2024.
System updates and performance
In ORAS6, the ocean and sea-ice model has been updated and is now driven by hourly ERA5 forcings. A novel ensemble-based variational data assimilation system has been developed that provides flow-dependent background error variances and vertical correlation scales. Other updates include the assimilation of level-4 (L4) SST and level-3 (L3) sea-ice concentration data, an improved ensemble generation method, and updates in the bias correction scheme and altimeter data assimilation. Figure 1 is a schematic plot of the ORAS6 system, highlighting major system improvements compared to ORAS5.
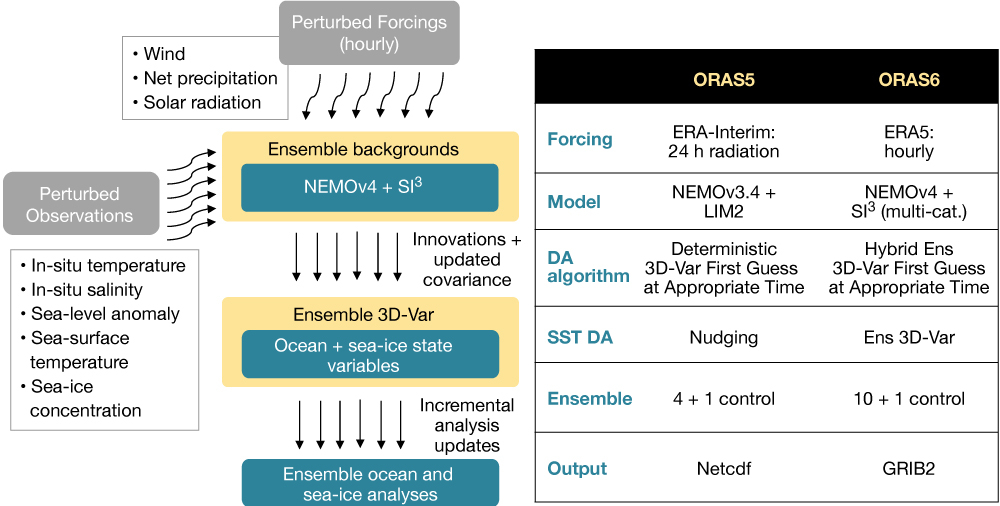
Summary of ocean and sea-ice model updates
The ocean and sea-ice model is now based on NEMOv4.0.6, which includes a new multicategory sea-ice model SI3, with prognostic salinity. This is a step change in modelling capability for sea ice. The assimilation system has had to be adapted to account for new prognostic variables in the sea ice, as well as for the concept of representing the ice with multiple thickness categories. The ocean model itself has also changed by extending the southerly limits of the grid around Antarctica, and by modifying the way atmospheric forcing is applied to the ocean in a standalone model (i.e. not coupled to an overlying atmosphere). The resolution of the ocean remains unchanged at ¼ degree and with 75 vertical levels. The forcing is applied hourly from ERA5 and has a new bulk formulation for the computation of fluxes that is much closer to the ECMWF operational forecasting system. Thanks to this hourly ERA5 forcing and switching to direct assimilation of SST observations, ORAS6 represents SST with a diurnal cycle closely matching that reconstructed from drifter data (see Figure 2). More details about the model developments can be found in the NEMOv4 article in this Newsletter.
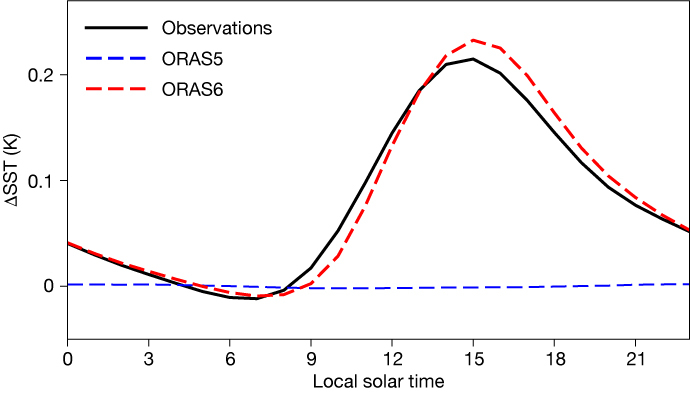
SST data assimilation with Ocean EDA system
Direct data assimilation of L4 SST data has been introduced in ORAS6. One major challenge in SST data assimilation is that the background error covariance formulation used in ORAS5 is not optimised to deal with very dense satellite SST observations together with a sparse ocean in-situ observing network. To solve that, ORAS6 features deployment of an Ensemble of Data Assimilations successfully developed by ECMWF in collaboration with Member State institutes. This was done in the framework of a contract of the EU-funded Copernicus Climate Change Service (C3S) implemented by ECMWF (Chrust et al., 2021). The hybrid background error covariance matrix is a key element of the data assimilation system, determining the spatial structure of corrections to initial conditions arising from observations. It combines newly computed seasonally varying climatological variances and horizontal correlation length scales with flow-dependent errors of the day and, most importantly, vertical length scales estimated from an ensemble. The latter was enabled by an innovative normalisation algorithm and is crucial for effective assimilation of surface observations. A new version of a reprocessed OSTIA L4 SST product from the UK Met Office is assimilated in ORAS6 to ensure temporal consistency to its homonymous real-time product. It has been found that, compared to SST nudging, direct assimilation of SST data with this new ocean EDA system has greatly reduced SST biases, especially in Gulf Stream regions to the north of Cape Hatteras, where the ¼ degree NEMO model has a persistent warm water over-shooting issue. Figure 3 shows SST biases in the Gulf Stream region, with clearly superior performance of ORAS6 compared to its predecessor.
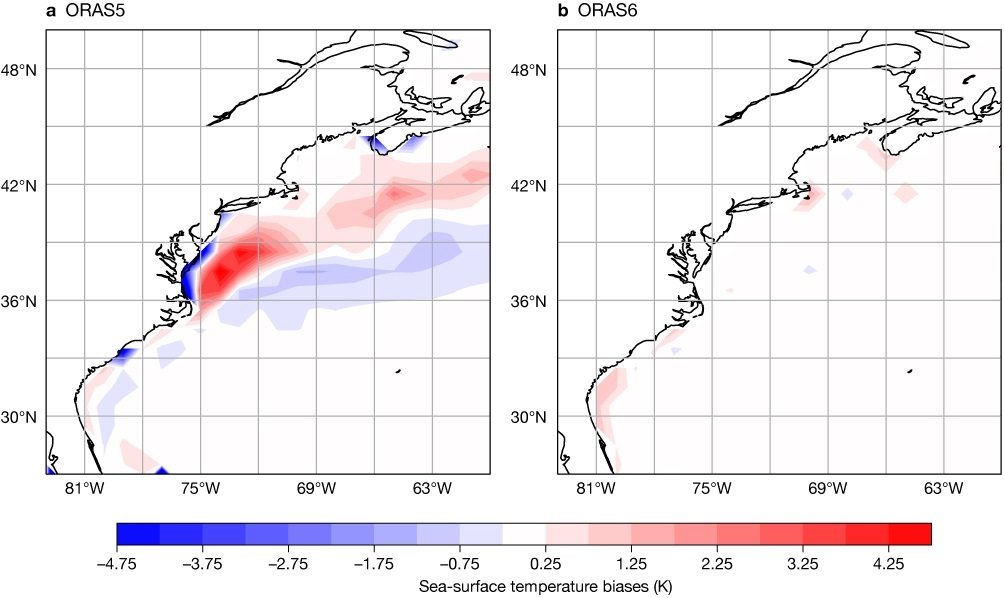
Sea-ice data assimilation with SI3
Many developments were required to successfully use data assimilation with the new multicategory SI3 model. Care has been taken to ensure the application of single category concentration increments into the multicategory model does not unduly change the model ice thickness. A balance between sea-ice concentration and sea-water temperature was introduced to enable the model to retain concentration increments and in turn to provide a better fit to observations. ORAS6 assimilates the latest version of the OSI SAF global sea-ice concentration climate data record (L3) from EUMETSAT, based on observations from three satellite instruments: the Special Sensor Microwave/Imager (SSM/I), the Special Sensor Microwave Imager/Sounder (SSMIS), and the Scanning Multichannel Microwave Radiometer (SMMR). New methods on observation perturbations, and a way to account for observation error variances using uncertainty information, have been developed in order to assimilate L3 sea-ice data. Compared to gridded OSTIA L4 sea-ice concentration data used by ORAS5, switching to L3 OSI SAF data could potentially increase our operational reliability by making our ocean and sea‑ice analysis less susceptible to errors in external data production (e.g. data infilling method). The overall performance of sea-ice concentration in ORAS6 is improved when compared to ORAS5 (see Figure 4). Sea-ice thickness properties in ORAS6 have changed substantially due to differences in forcing and the sea-ice model. In some regions of the Arctic, the sea ice in ORAS6 can be biased thin. Attempts to constrain sea‑ice thickness in ORAS6 have been made, including nudging towards a merged sea-ice thickness reconstructed dataset from a product based on observations of ESA's CryoSat2 and SMOS satellite instruments (CS2SMOS). This approach was not pursued mainly due to the product only being available in a fairly recent period (from 2011 on), and also due to discrepancies between this thickness product and the assimilated OSI SAF sea-ice concentration product.
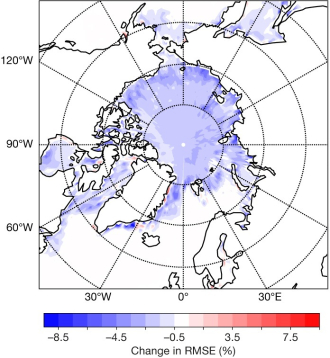
Update on ensemble generation
Sampling the uncertainty in the ocean state is important to provide a reliable ensemble of i) ocean initial conditions for coupled forecasts from the medium range to the seasonal range, and ii) lower atmospheric boundary conditions over the ocean and sea-ice surface for the upcoming ERA6 reanalysis. Reliable model background ensemble variance is also critical in the ocean EDA system in estimating flow-dependent errors of the day. Many new developments have been implemented in the ORAS6 ensemble generation so perturbation can be applied to the newly introduced SST and L3 sea-ice concentration assimilation. ORAS6 uses surface flux forcing from the ERA5 reanalysis. Uncertainty of air–sea fluxes is known to be high and needs to be accounted for in an ensemble of analysis. The perturbation of the sea-surface forcing is based on a pre-built repository that includes perturbations of wind stress, SST, sea-ice concentration (SIC), freshwater and heat fluxes. It accounts for differences between separate atmospheric reanalysis datasets (Structural Errors – SE), and for uncertainties by sampling all individual analysis members from the same atmospheric reanalysis product (Analysis Errors – AE). In ORAS6, SE are computed for the wind stress using monthly outputs from ERA5 and the Japanese 55-year reanalysis (JRA-55), and for SST and SIC using monthly fields from OSTIA reprocessed analyses and ESA-CCIv2 analyses. AE are computed for SST, evaporation-minus-precipitation (EMP), solar radiation, and wind stress, using all ERA5 ensemble members. The two types of error are considered independent and thus additive. The perturbation repository is stratified by calendar month and date range to capture uncertainties that correspond to the season and the level of sampling of the ocean by the observing system. In addition to surface forcing perturbations, the same perturbations on ocean in-situ observations as in ORAS5 are also activated in ORAS6, including additional stratified random sampling when assimilating SST observations. Figure 5 shows that the ORAS6 SST ensemble spread is generally larger than that estimated from its predecessor product, thanks to this updated ensemble generation scheme.
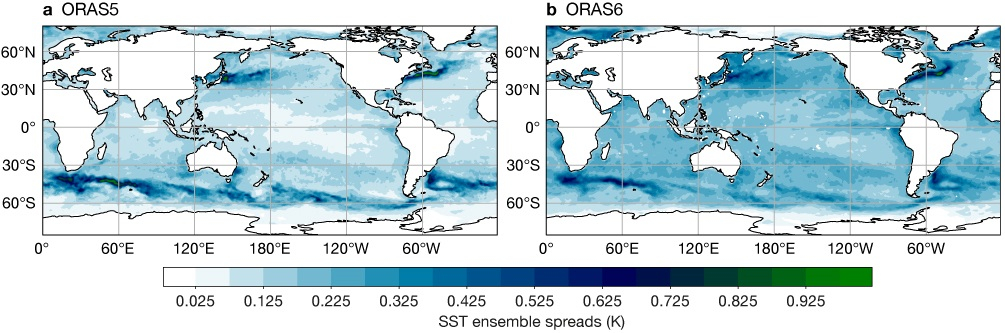
Other ocean data assimilation system updates
Sea-surface height (SSH) assimilation in ORAS6 has seen several major updates as well. Compared to ORAS5, ORAS6 assimilates a new reprocessed Sea Level Anomaly (SLA) dataset from the multi-satellite Data Unification and Altimeter Combination System (DUACS), made available through the Copernicus Marine Environment Monitoring Service (CMEMS). This provides altimeter data from more satellites with improved temporal consistency. Unlike in ORAS5, SLA data in high latitudes (outside a latitude band from 50°S to 50°N) and near continental coasts are also assimilated in ORAS6, thanks to reduced regional biases in this new SLA product. As a result, SSH in ORAS6 is much improved compared to ORAS5, especially in regions with additional altimeter data assimilation (see Figure 6). We continue to use a model-based estimation of Mean Dynamic Topography (MDT), which is part of the forward model in altimeter assimilation. While errors in MDT are not explicitly accounted for in the ocean EDA cost function, we have introduced new methods to mitigate biases in MDT, namely a two-step approach and removal of SSH trend errors in pre-production runs used to estimate MDT.
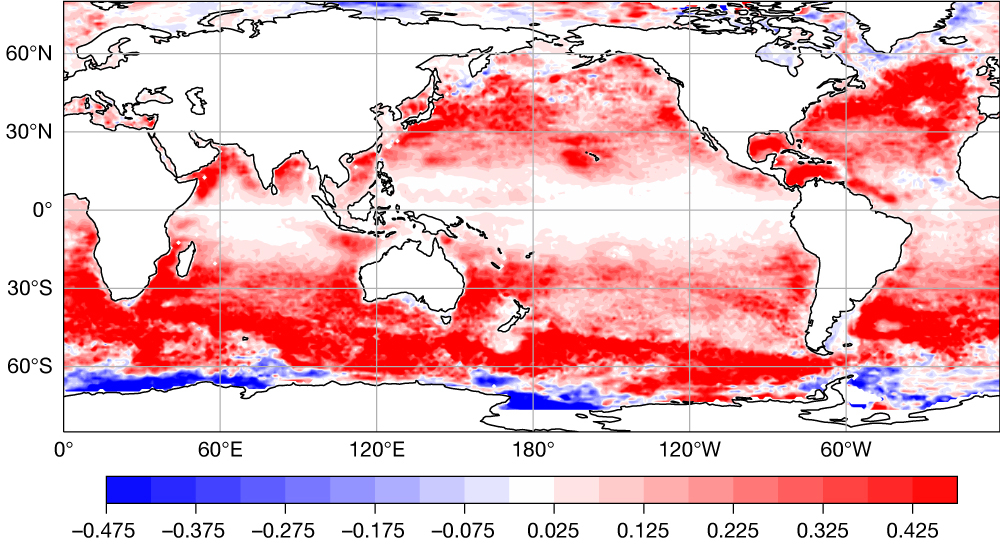
The model bias correction scheme implemented in ORAS6 still comprises a priori bias and online bias to account for biases with different spatial and temporal scales. Compared to ORAS5, the a priori bias terms in ORAS6 are estimated with a multi-step approach, including an additional spatial filter. We have also introduced a new vertical element in addition to the original 2D partition coefficients matrix, which is used to specify partition of the assimilation increments into the different bias control variables. A new analytical function has also been introduced to compute partition coefficients for the online bias correction term. These new developments are required for the model bias correction scheme to work successfully together with other new ORAS6 elements, such as the ocean EDA and direct assimilation of SST observations.
We have introduced a new method to distribute freshwater budget (FWB) adjustments in ORAS6, in which global water mass and volume adjustments are distributed according to the model background EMP value, by separating regions with positive EMP from those with negative EMP.
Forecast performance with ORAS6
To evaluate the medium-range forecast performance for predicting SSTs, we used a quality- controlled dataset from the Global Drifter Program of the US National Oceanic and Atmospheric Administration (NOAA) (https://www.aoml.noaa.gov/phod/gdp/). Figure 7 shows global change in root-mean-square error (RMSE) with the prototype ORAS6 system compared to the operational ORAS5 system for a one-year period. The new system reduces the error in SST almost everywhere, with improvements being larger in boundary current regions and in the Southern Ocean. Results from testing with a copy of the operational forecasting system with an atmospheric resolution of 9 km (medium-range ensemble resolution) show that the improvement is maintained for longer lead times until day 9 (see Figure 8). Comparing the system performances at different lead times shows that errors in SST predictions at day three of the new system are comparable with those of the old ocean analysis system.
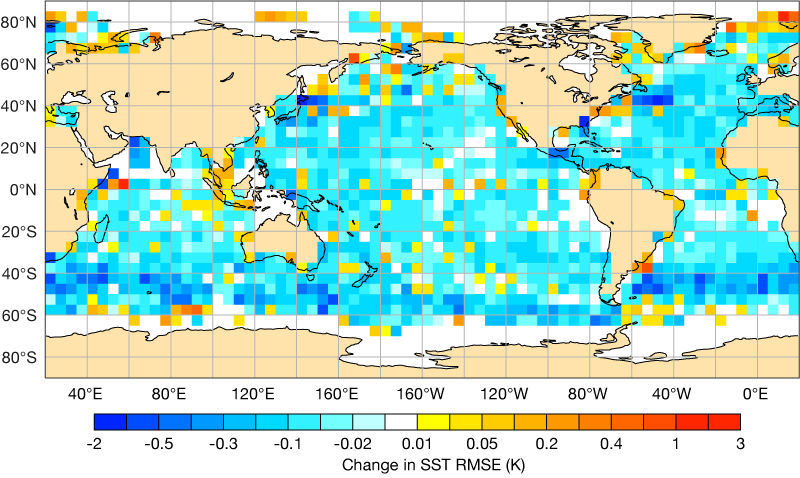
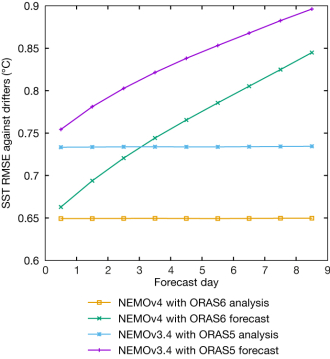
Conclusion
In summary, the design and development of ORAS6 has been guided by the following criteria:
- to maintain the climate quality required for its existing applications
- to improve the representation of the surface of the ocean to cater for the needs of medium-range forecasts and upcoming coupled atmospheric reanalyses
- to use the latest scientific developments and modelling tools, so the operational system and its users can benefit from years of research
- to facilitate data access to both external and internal users, as well as long-term data curation.
ORAS6 will span the period from 1950 to the present. To facilitate its timely production and to account for the evolving nature of the global ocean observing system, ORAS6 is being produced in separate parallel streams, which are connected via a continuous prior analysis to ensure there is climate continuity. At the time of writing, the operational production of the most recent ORAS6 stream (2005 to the present) is under way, and work is ongoing on preparing the settings for the early and pre-satellite streams. It is expected that the whole ORAS6 record will be completed by the end of 2024, and data should be available for external users shortly after. ORAS6 SST and sea-ice will be used as lower boundary conditions for ERA6. This has been possible thanks to the improvement in SSTs, especially in western boundary currents and the representation of the diurnal cycle. ORAS6 will also be used to initialise the re-forecasts of the upcoming seasonal forecasting system, SEAS6, and it will form the basis for the initialisation of the forecasts for Cycle 50r1, which will be implemented in 2025.
The priority given to surface fields in ORAS6 is in agreement with ECMWF’s strategy to exploit interface observations. It is a first step to enable further advances with coupled data assimilation (de Rosnay et al., 2022). ORAS6 will also provide a relevant dataset for emerging data-driven models of the ocean and sea ice.
In the longer term, future generations of ocean reanalysis will be conducted at higher spatial resolution, with potential 4D-Var approaches to further improve the assimilation of SST and other surface observations. This will ideally make it possible to provide smoother and more consistent climate data records. We also intend to devote efforts to the assimilation of more interface observations, such as sea-ice thickness and snow-over-sea-ice information, which have proven to be sources of predictability across timescales.
Acknowledgement
We acknowledge the contributions of a C3S tender (C3S_321b) for the development of the EDA in the NEMOVAR system. Contributions were made by the National Institute for Research in Digital Science and Technology (Inria, France), the Centre Européen de Recherche et de Formation Avancée en Calcul Scientifique (CERFACS, France), the Centre for Maritime Research and Experimentation (CMRE, Italy), and the Met Office (UK).
The NEMOv4 and SI3 versions have been provided by the NEMO community model https://www.nemo-ocean.eu/. The specific configurations used in ORAS6 have benefited from interactions with the UK Joint Marine Modelling Programme.
The development of the ORAS6 system was partially supported by the CMEMS GLORAN project (third phase) under contract number 21003-COP-GLORAN-LOT3.
Further reading
Zuo, H., M.A. Balmaseda, S. Tietsche, K. Mogensen & M. Mayer, 2019: The ECMWF operational ensemble reanalysis–analysis system for ocean and sea ice: a description of the system and assessment. Ocean Science, 15(3), 1–44. https://doi.org/10.5194/os-15-779-2019
Chrust, M., M.A. Balmaseda, P. Browne, M. Martin, A. Storto, A. Vidard et al., 2021: Ensemble of Data Assimilations in the ocean for better exploitation of surface observations. ECMWF Newsletter No. 168, 6–7. https://www.ecmwf.int/en/newsletter/168/news/ensemble-data-assimilations-ocean-better-exploitation-surface-observations
Sármány, D., K. Mogensen, M. Valentini, R. Aguridan, P. Geier, J. Hawkes et al., 2024: New software libraries for ORAS6 ocean reanalysis. ECMWF Newsletter No. 178, 13–15. https://www.ecmwf.int/en/newsletter/178/news/new-software-libraries-oras6-ocean-reanalysis
de Rosnay, P., P. Browne, E. de Boisséson, D. Fairbairn, Y. Hirahara, K. Ochi et al., 2022: Coupled data assimilation at ECMWF: current status, challenges and future developments. Quarterly Journal of the Royal Meteorological Society, 148(747), 2672–2702. https://doi.org/10.1002/qj.4330