In a significant milestone, ECMWF has implemented the first operational data-driven forecasting system, the AIFS (Artificial Intelligence Forecasting System), demonstrating the Centre’s commitment to pursue the best possible weather forecasts with both physics-based and machine learning (ML) methods. There has been an explosion in scientific publications featuring ML‑based forecasting systems over recent years. The vast majority of these systems depend on ECMWF's ERA5 reanalysis dataset and Integrated Forecasting System (IFS) operational analysis for training and initialisation of their forecasts. They have showcased how traditional physics-based numerical weather prediction (NWP) forecast models are outperformed for a number of headline scores at a much-reduced computational cost. Now this technology has been leveraged for operational meteorology with the implementation of the AIFS Single. This first version, AIFS Single 1.0, is named to highlight that it produces a single forecast, to be complemented later this year with a first AIFS ensemble system. Having implemented the AIFS Single, we can now begin to understand its performance at an event-based level with feedback from forecasters, as it runs operationally alongside the IFS.
Basic characteristics
The AIFS Single has an encoder–processor–decoder structure, where the encoder first projects the model state onto a lower-resolution grid, using a graph neural network (GNN). The processor then uses a Transformer to update the model state 6‑hours in time before the decoder reprojects the model state back to the target resolution. Further details on the model structure can be found in Lang et al., 2024a (https://arxiv.org/abs/2406.01465). The AIFS Single 1.0 was trained on ECMWF’s ERA5 reanalysis dataset (1979–2022) and then fine-tuned to the IFS operational analysis (2016–2022).
The AIFS Single is now fully built under the open-source Anemoi framework developed by ECMWF and its Member States (see ECMWF Newsletter No. 181: https://tinyurl.com/bdh5bc5a). This ensures the development, training and implementation of such data-driven models are reproducible, traceable and scalable. More information on the Anemoi framework can be found within the set of Anemoi webinars (https://events.ecmwf.int/event/446/). The AIFS Single 1.0 has been released as a model on the ML model-sharing platform Hugging Face, including a guide on how to use this from ECMWF’s open data stream (https://huggingface.co/ecmwf/aifs-single-1.0).
New features
The AIFS Single has expanded the number of forecast variables compared with other data-driven systems. These include solar radiation and 100 m wind speeds, useful for energy forecasting and pricing. We now include cloud cover, snowfall and additional land surface variables of soil temperature, soil moisture and runoff. The physical consistency of these new parameters can be seen in the figure, where the model has an internally consistent representation of clouds for total cloud cover and solar radiation at the surface. Some of these new variables can already be explored through ECMWF charts (https://charts.ecmwf.int/?query=aifs-single).
We have increased the physical consistency of the model by including ‘boundings’ as an additional model layer after the decoder. We explicitly bound total precipitation, specific humidity, runoff and solar radiation by zero. Soil moisture and total cloud cover are bounded between zero and one. Snowfall, convective precipitation and low/medium/high cloud cover are bound to be fractions of total precipitation and total cloud cover respectively. This has led to increased forecast scores for precipitation and reduced previous issues, where it was noted the model produced an excess of light rainfall.
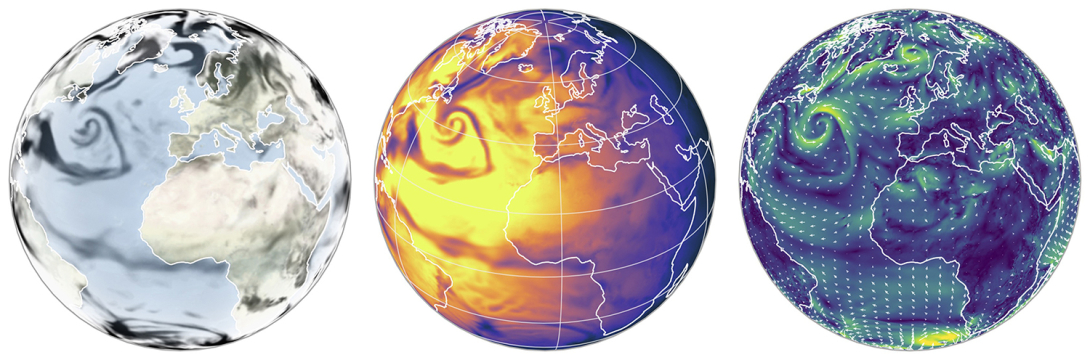
Early performance
The AIFS Single is already showing strong performance while running operationally, including forecasting rare events that have no clear analogies in the model’s training data. This can be seen clearly in the extreme snow event over the Gulf Coast of America on 21 January 2025 (see the second figure). This had a return period of roughly 100 years, i.e. such events are very rare or do not appear at all in the ERA5 reanalysis or the operational archive. These types of events provide confidence that the model is not only reproducing its training data but has learnt a representation of atmospheric physics. The ability of an earlier AIFS to capture extremes is also noted by Ben Bouallègue et al., 2024 (https://doi.org/10.1175/BAMS-D-23-0162.1). In general, the AIFS Single, due to training to minimise the mean squared error, still struggles to represent small-scale extreme events. First steps to address this will be made with the upcoming AIFS ensemble system.
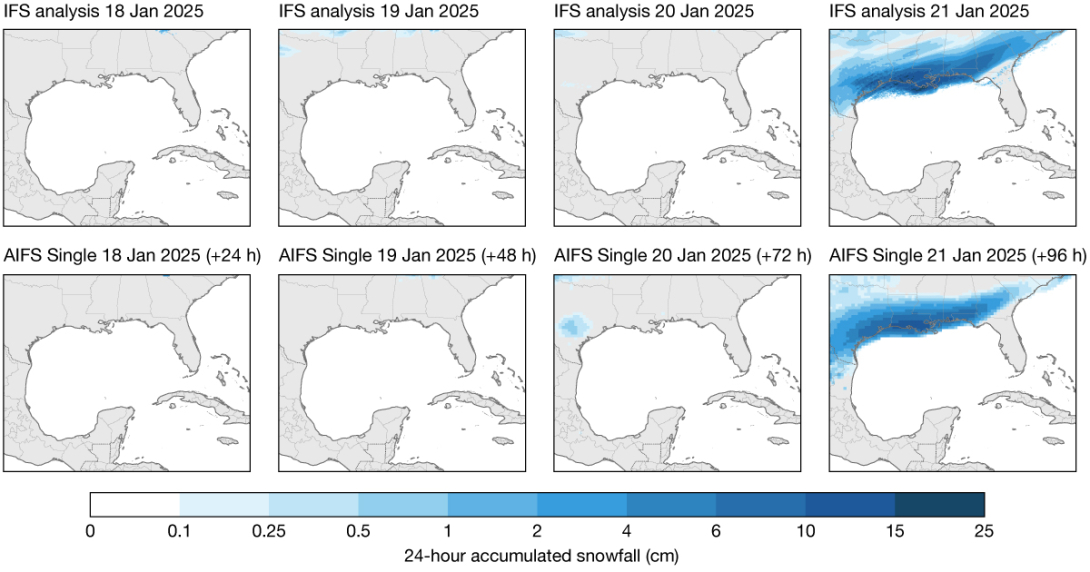
Outlook
The operational release of the AIFS Single has been the culmination of a lot of work across ECMWF. That includes work from the start of the process – by those who contribute to the creation of the ERA5 reanalysis and IFS operational analysis, vital to train and initialise the AIFS – to the end – by those who integrate AIFS models into real-time production and dissemination pipelines for forecasts.
New developments are already in progress for the AIFS. An ensemble version of the AIFS, trained using a Continuous Ranked Probability Score (Lang et al., 2024b: https://arxiv.org/abs/2412.15832), is targeted for operational release in the summer of 2025. Work is also under way to ‘fine-tune’ the ~28 km AIFS Single towards the IFS operational resolution of ~9 km, making full use of operational data, which is currently only used by the AIFS at ~28 km resolution. Within the EU’s Destination Earth initiative and the Copernicus programme, to which ECMWF contributes, more Earth system components are being actively trained using ML methods, including waves, the ocean, sea ice, atmospheric composition, and hydrology.