A rich stream of never-seen-before cloud and aerosol observations has begun flowing through the ECMWF data centre. Back in 2024, on a fittingly cloud-filled Californian May morning, the EarthCARE (Cloud, Aerosol and Radiation Explorer) satellite was launched into space from Vandenberg Space Force Base. Due to its size, a SpaceX Falcon 9 rocket was used to deliver EarthCARE into a sun-synchronous orbit at an altitude of 400 km. EarthCARE is the 8th European Space Agency (ESA) Earth Explorer mission and a joint venture between ESA and the Japan Aerospace Exploration Agency (JAXA). Equipped with four synergistic instruments – a Doppler radar, a high-spectral-resolution lidar, a multi-spectral imager and a broadband radiometer – EarthCARE provides unprecedented insights into the interactions between clouds, aerosols, precipitation and radiation. These processes play a key role in the Earth’s weather and climate, yet they are some of the least understood. As greenhouse gas concentrations continue to rise, and severe weather is projected to become more frequent and intense, high-quality observations are essential for improving our understanding of the atmosphere and improving our climate and numerical weather prediction models.
ECMWF’s forecast model, like many other models, exhibits regional biases in clouds and their radiative effects. These inaccuracies lead to biases in temperature and winds that affect the fidelity of ECMWF’s medium-range forecasts. The biases become even more important for seasonal prediction, which requires a model to be as free of ‘drifts’ as possible. EarthCARE offers new observations to help pinpoint where our model is going wrong – not just in cloud microphysical properties, such as rain drop size and cloud water amount, but also something that has never been quantified from space before: the fall-speed of snow and rain. These much-anticipated observations are already being used to evaluate the cloud physics within ECMWF’s Integrated Forecasting System (IFS).
For NWP, EarthCARE offers a second, more direct benefit through its use in data assimilation. The 4D‑Var assimilation of humidity- and cloud-sensitive observations at ECMWF to improve the initial conditions of forecasts has increased dramatically over the past decade. This has been driven by advances in ‘all-sky’ microwave radiance techniques, improvements in cloud representation within both the forecast model and the data assimilation system, and a growing number of meteorological satellites in orbit. Including active remote sensing measurements of clouds and precipitation from EarthCARE could further enhance the initial conditions produced from ECMWF’s assimilation system.
In 2016, work began to enable the direct assimilation of space-borne radar and lidar observations within the ECMWF 4D-Var system. Once complete, using CloudSat and CALIPSO data as proxies for EarthCARE’s radar and lidar, tests demonstrated a direct impact on forecast skill (Janisková & Fielding, 2020). Assimilating radar reflectivity and lidar backscatter improved largescale temperature and wind forecasts, as well as the analysis of clouds and precipitation. While CloudSat and CALIPSO were never considered operational missions, their influence on data assimilation highlighted the potential value of EarthCARE. This is why EarthCARE’s data is being made available in near real-time (often within two hours of observation), unlocking its potential for operational forecasting. In this article, we focus on the impact of EarthCARE on data assimilation in NWP.
Box A contains an overview of the instruments carried by EarthCARE and the measurements they provide.
A
EarthCARE leaves clouds and aerosols with nowhere to hide
The mission’s primary goal is to provide so-called ‘radiation closure’ for NWP and climate models. This means that the top-of-atmosphere radiative fluxes, which are measured by EarthCARE, can be predicted based on EarthCARE retrievals of the clouds, aerosols and precipitation underneath. To achieve this, four complementary sensors are used (see Figure 1). Firstly, the ATmospheric LIDar (ATLID), a UV high spectral resolution lidar (HSRL), is sensitive to aerosols and clouds, but is quickly attenuated in liquid cloud. By measuring the spectrum of backscattered laser to distinguish signals from particles and the air, it unambiguously retrieves the optical depth of clouds and aerosol within each lidar range bin.
Figure 2 showcases ATLID’s capabilities with an example of the total attenuated particulate backscatter. Over northeast England, a distinct aerosol layer at 6 km resides above isolated cumuli at 2 km. Further south are multiple layers of altocumulus, with the lower layer being capped by a thin layer of supercooled liquid water that fully attenuates the lidar signal.
To reveal cloud structure further down, the W‑band cloud profiling radar (CPR) is used. The CPR measures the backscatter from radar pulses that are sensitive to cloud and precipitation drops with a radius of around 10 μm and larger. Areas of greater reflectivity indicate larger and/or more numerous particles. Looking again at the altocumulus in Figure 2, we can now see snow underneath the supercooled liquid layer, likely formed through rapid vapour deposition onto ice crystals where the air is super-saturated with respect to ice. Part of the cloud has exhausted its supercooled liquid supply, but has precipitation falling to an altitude of 4 km.
As an additional constraint on the particle size of clouds and aerosols, a Moderate Spectral-resolution Imager (MSI) observes radiances across several different wavelengths in the visible and near-infrared. The bottom panel in Figure 2 shows how the different wavelengths can also be used to separate liquid (white) and ice (blue) clouds. Finally, to verify the mission goal of radiation closure, a broadband radiometer (BBR) observes broadband radiative fluxes at three different viewing angles along the EarthCARE track.
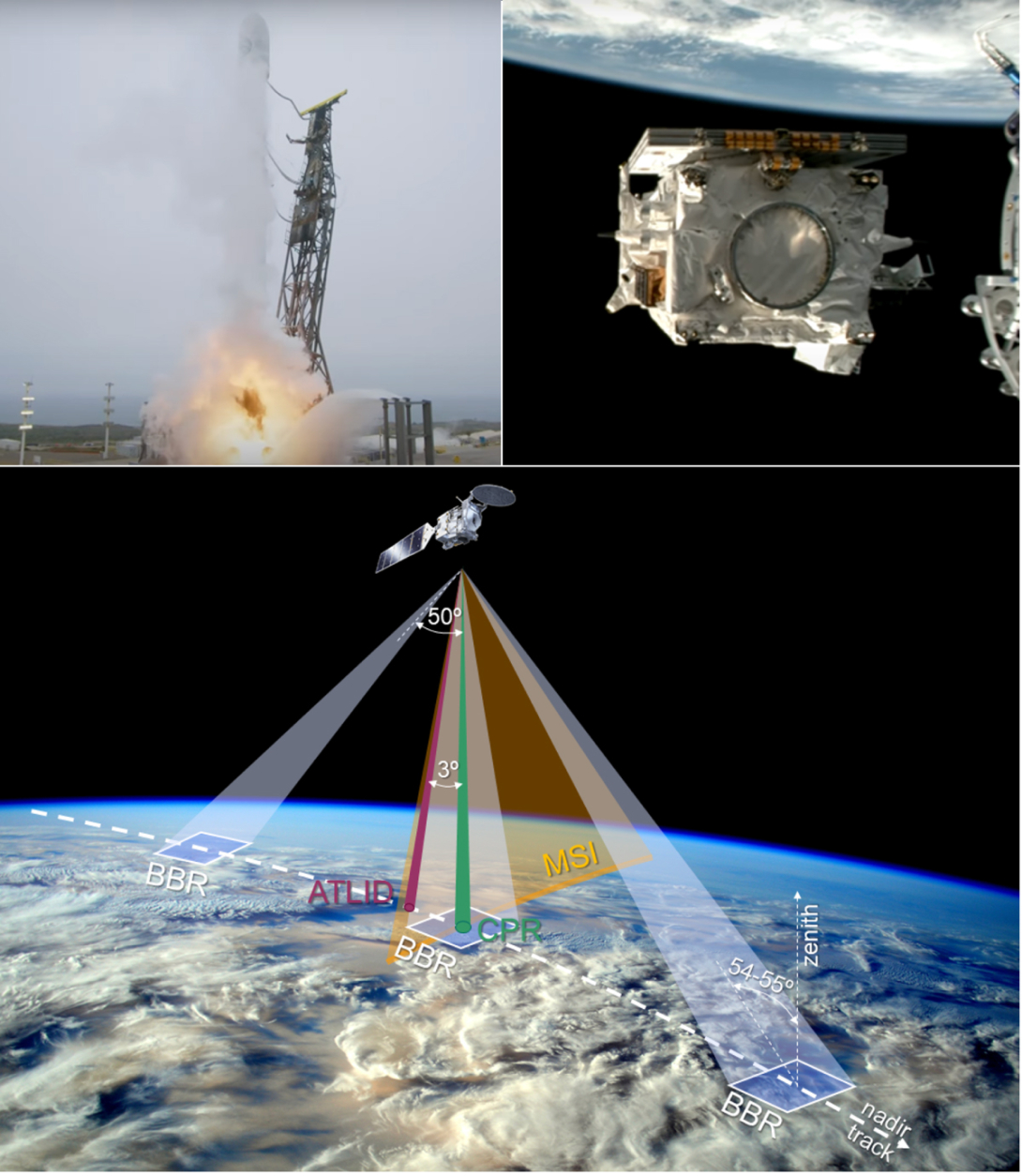
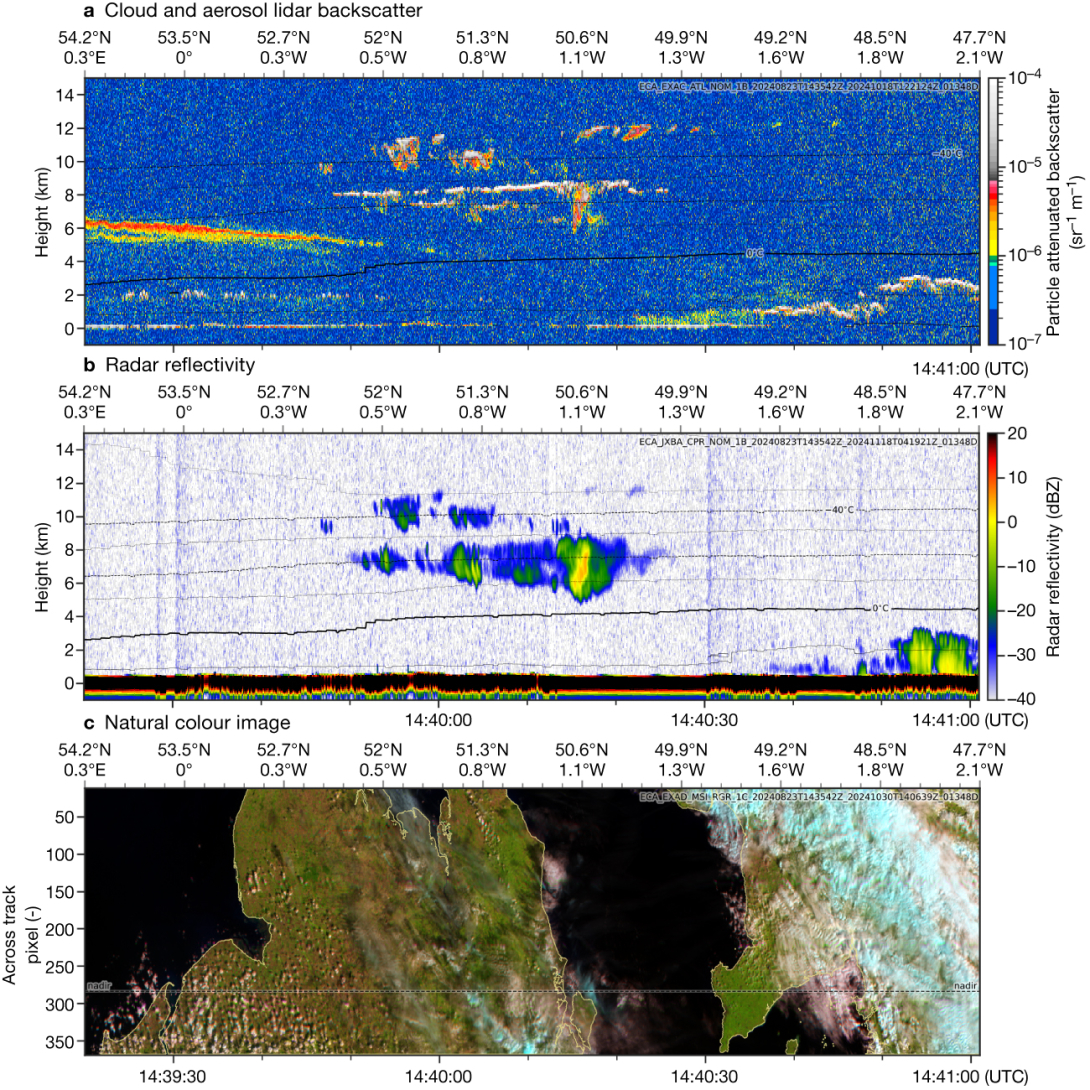
Taking all-sky assimilation to a new dimension
In contrast to all-sky microwave sounder observations, which have a broad sensitivity to the vertical profile of temperature and humidity, radar and lidar observations observe the atmosphere layer-by-layer with much higher resolution. The complex structure of clouds that is revealed is a mixed blessing when it comes to using the observations in data assimilation. While the new observations contain a huge amount of information on the atmospheric state, the 4D‑Var data assimilation system at ECMWF fundamentally assumes a linear relationship between changes in the measurement (e.g. radar reflectivity) and changes in model state variables (such as temperature and humidity). If the model state is too far from the observations, this linearity assumption will be invalid and will lead to a sub‑optimal minimisation and analysis. To mitigate these challenges, a raft of new developments was needed.
A new forward model to map from model space to observation space was implemented. By taking a ‘triple-column’ approach, sub‑grid variability in cloud cover and cloud amount is accounted for by simulating the radar and lidar signal passing between three sub-columns. For screening, observations that have large first-guess departures (large differences between short-range forecasts and observations) are discarded. This is also done where there is no cloud or precipitation in the model. There are also situations where we know our forward model does not account for multiple scattering of the radar or lidar signal, so cases where this is suspected, such as in the cores of deep convection, are also excluded.
Another important aspect of data assimilation is quantifying observation errors, as they weight the influence of an observation. Observation errors of cloud measurements are highly regime dependent. To estimate how much weight to give to the EarthCARE observations within 4D‑Var, we therefore take an ‘error inventory’ approach that adds different sources of error in quadrature. Often the sampling error resulting from the radar’s narrow footprint relative to the size of grid boxes dominates. However, in some situations, such as stratiform ice cloud with relatively little turbulence, the uncertainties in certain microphysical assumptions can be larger.
ECMWF’s role in the commissioning phase
Before incorporating new observations into ECMWF’s assimilation system, the measurements must be carefully monitored for consistency and stability, while also checking for biases or artifacts that could degrade the analysis. Observation monitoring at ECMWF is also invaluable for the calibration and validation of satellite instruments. Comparing observational and model data significantly enhances the detection of sudden jumps or drifts in bias.
For example, ECMWF’s monitoring was instrumental in identifying and correcting mirror-temperature-dependent biases in ESA’s seventh Earth Explorer, Aeolus. To support EarthCARE’s calibration and validation activities, ECMWF began routine monitoring of CPR and ATLID data within days of the instruments being activated.
During EarthCARE’s commissioning phase, NWP monitoring was particularly useful for tracking the radar calibration. Jumps in calibration due to changes in the signal processing were quickly reported to ESA and JAXA, which proved invaluable for helping engineers to troubleshoot problems. Traditional methods to calibrate space-borne radar rely on either direct overpasses with calibrated sensors on the ground, or a statistical analysis of sea‑surface returns, both of which require many days to complete. Averaged statistics using ECMWF’s OBSTAT plotting software (Figure 3) have also been useful for monitoring the radar and lidar observations. In Figure 3, the mean first-guess departures for Rayleigh backscatter reveal some contamination with spurious signals over Russia, the presence of Arctic polar stratospheric clouds not represented in the model, and upper tropospheric aerosols in the tropics (a negative bias due to the lack of attenuation in the model).
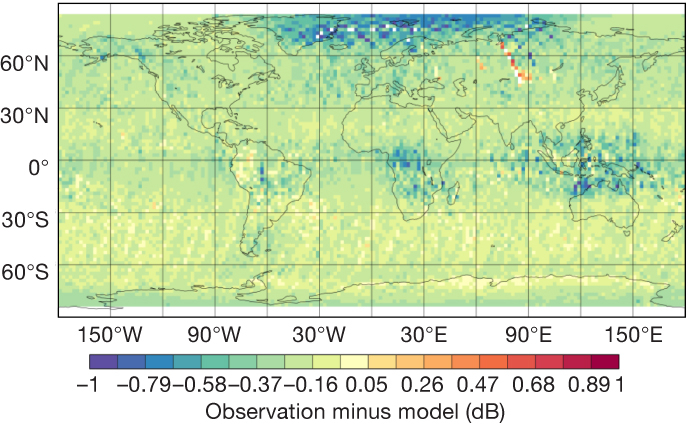
Early assimilation tests and impact on forecasts
Preparations for radar and lidar assimilation during the joint ESA–ECMWF ‘PEARL Cloud’ project enabled EarthCARE assimilation experiments to begin soon after launch. Figure 4 shows an example of the assimilation of total attenuated backscatter for a section of an EarthCARE orbit over the tropical West Pacific, beginning over Australia and then passing Papua New Guinea. The frame is dominated by high ice cloud near the tropopause associated with the outflow from convection and a broad area of active deep convection at 10°N, which fully attenuates the lidar signal. Before assimilation (in the first-guess panel), much of the active convection in the model is displaced compared to the observations. However, after assimilation (in the analysis panel), the model represents the cloud positions much better. The mid‑level convection over Papua New Guinea is also better represented.
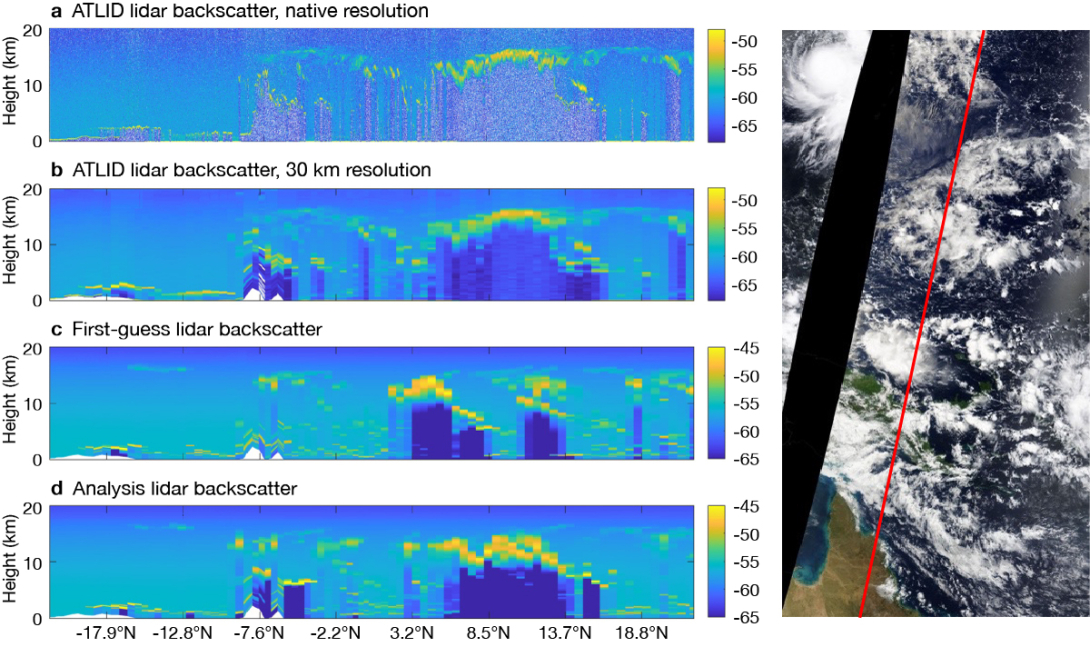
A demonstration of the assimilation of CPR radar reflectivity is shown in Figure 5. In this case, EarthCARE passed over Libya before traversing central Europe and Sweden. An occluded front lay across much of France and Germany, as seen by the heavy precipitation in the radar around 50°N. Assimilating the observations helps move the front further south in the analysis. Cloud amount in the southern part of the front is also improved by the assimilation.
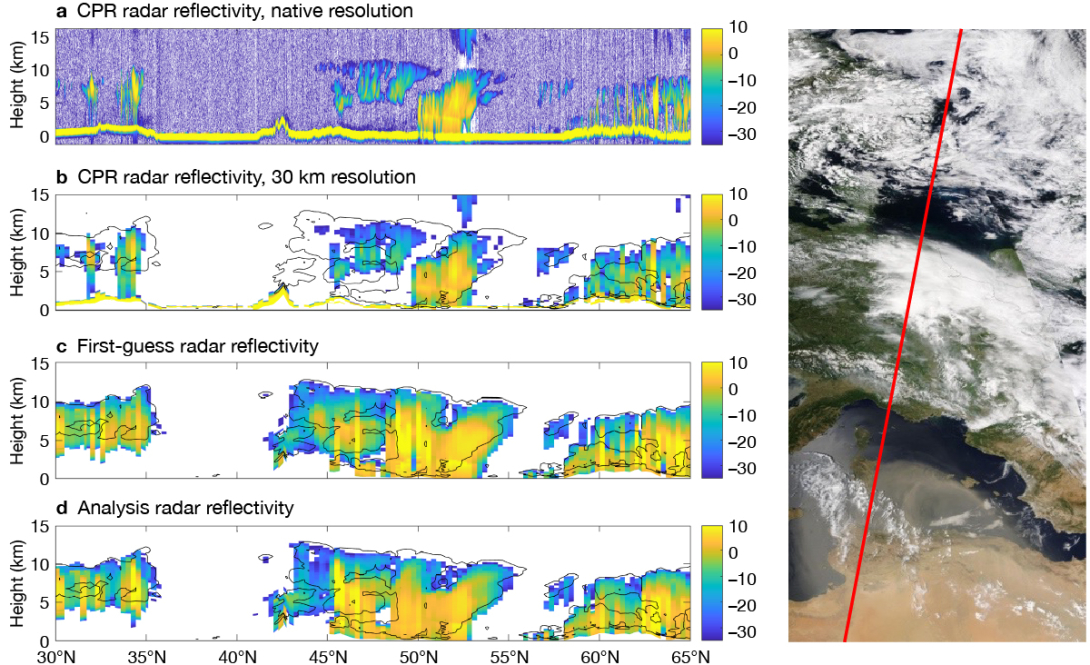
In addition to assimilation case studies, the impact of assimilating EarthCARE on forecast skill has begun to be investigated. Two three-month cycling analysis experiments between August and October 2024 were performed, one where all regularly assimilated observations were used, and one where EarthCARE radar reflectivity and lidar attenuated backscatter observations were also included. Figure 6 shows that the change in root-mean-square error of vector wind relative to the control is improved in the northern hemisphere when assimilating EarthCARE, with some statistical significance beginning to appear at a forecast lead time of five days. Elsewhere, the impact is generally neutral, but these results are extremely encouraging for a new sensor, and further testing and tuning of observation errors and screening is likely to improve the impact. Longer experiments are also required to reach statistical significance (typically six‑month experiments are required to detect significant impacts for single sensors).
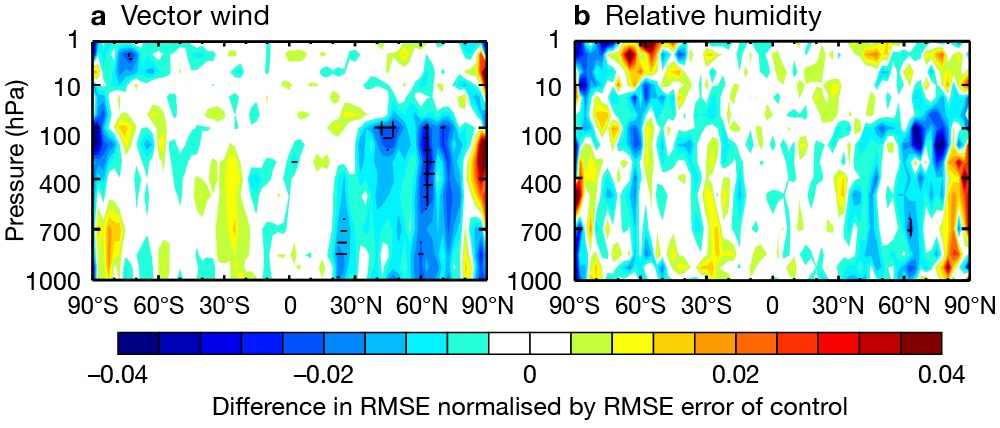
The future is bright
EarthCARE data will be included in the operational data assimilation as soon as possible, subject to the usual checks for including new observations. If all goes well, our forecasts will begin directly benefiting from EarthCARE this year. However, the use of EarthCARE at ECMWF will not end there. In a similar way to the cloud observation, aerosol signals from ATLID are planned to be included in operations of the EU’s Copernicus Atmosphere Monitoring Service (CAMS), implemented by ECMWF, within the next year. The HSRL’s capability to directly measure layer-by-layer aerosol radiative properties will offer a paradigm shift in constraining the vertical distribution of aerosols, as demonstrated in Figure 7.
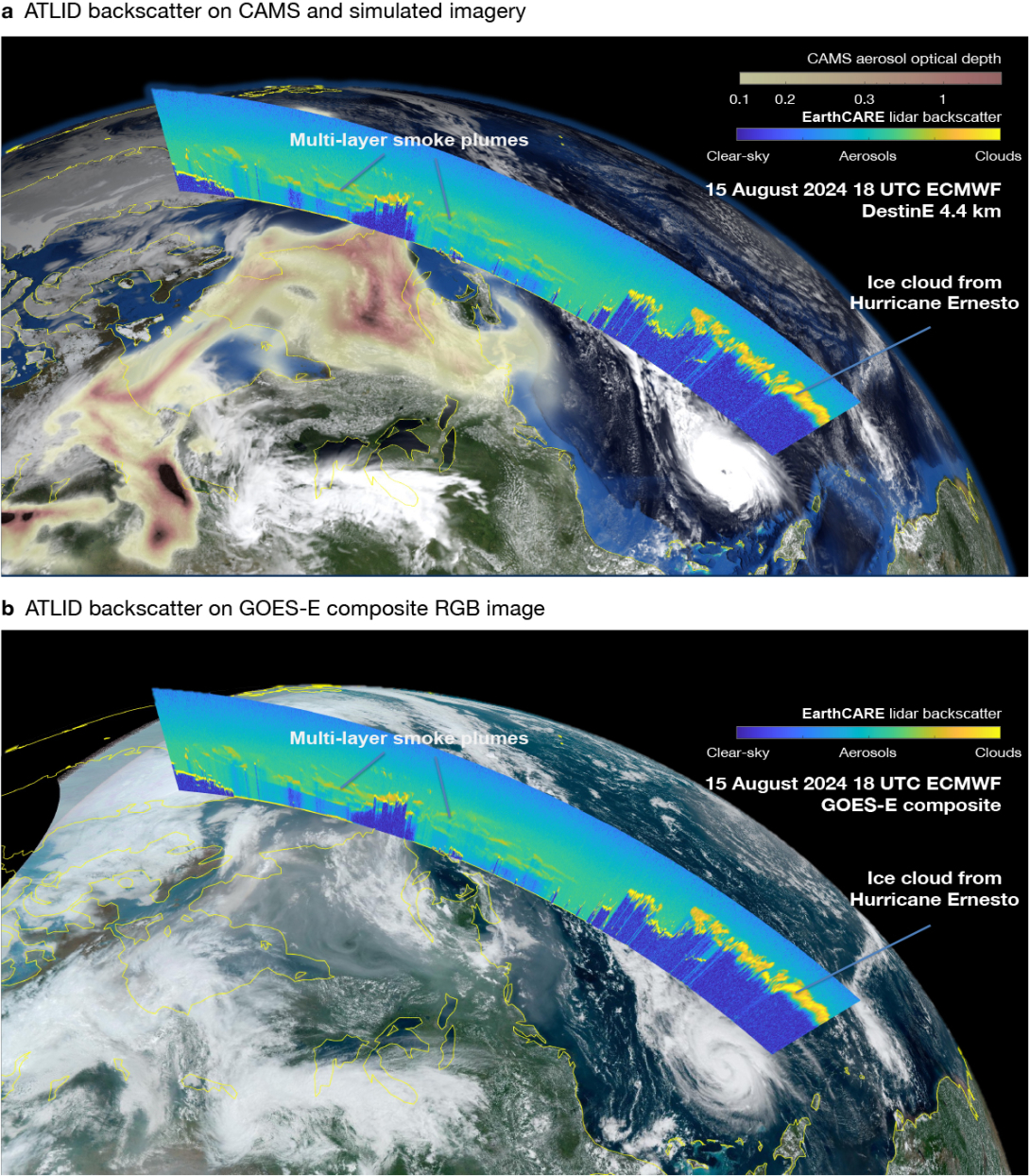
While this article has focused on the data assimilation of EarthCARE observations, the greatest impact is likely to come from using these observations to improve the physical processes within models. To assist with this, a unified retrieval to simultaneously retrieve aerosol, cloud and precipitation properties using three EarthCARE sensors has been developed at ECMWF. The products from this retrieval will be used by scientists in ECMWF Member States and worldwide to evaluate and improve NWP and climate models. The ESA-funded EarthCARE DISC (Data Innovation and Science Cluster) brings together scientists from many European institutes, including the Dutch national meteorological service (KNMI), the German Aerospace Center (DLR) and the National Observatory of Athens (NOA), to monitor, validate and improve this and other retrieval products.
For ECMWF, EarthCARE is perfectly aligned with our new Strategy 2025–2034, which aims for advances in km-scale modelling and Earth system development. The high-resolution observations of updrafts in convective cores have potential for evaluating the next generation of km-scale models. Using the Doppler radar, the parametrizations of ice and snow fall speeds in our model can be constrained on a global scale for the first time. The myriad interactions between aerosols and clouds can be elucidated, regional radiation biases deciphered, and drizzle evaporation rates quantified. All this will help drive down systematic model errors for improved forecasts. In short, watch this space for further exciting science from EarthCARE.
Further reading
Fielding M. D. & M. Janisková, 2020: Direct 4D-Var assimilation of space-borne cloud radar reflectivity and lidar backscatter. Part I: Observation operator and implementation, Q J R Meteorol Soc., 146, 3877–3899. https://doi.org/10.1002/qj.3878
Illingworth, A.J., H.W. Barker, A. Beljaars, M. Ceccaldi, H. Chepfer, N. Clerbaux et al., 2015: The EarthCARE Satellite: The Next Step Forward in Global Measurements of Clouds, Aerosols, Precipitation, and Radiation, Bull. Amer. Meteor. Soc., 96, 1311–1332, https://doi.org/10.1175/BAMS-D-12-00227.1
Janisková, M. & M.D. Fielding, 2020: Progress towards assimilating cloud radar and lidar observations, ECMWF Newsletter No. 162, 20–26. https://doi.org/10.21957/72xdt8mj03
Janisková, M. & M.D. Fielding, 2020: Direct 4D-Var assimilation of space-borne cloud radar and lidar observations. Part II: Impact on analysis and subsequent forecast. Q J R Meteorol Soc. 146, 3900–3916. https://doi.org/10.1002/qj.3879
Mason, S.L., R.J. Hogan, A. Bozzo & N.L. Pounder, 2023: A unified synergistic retrieval of clouds, aerosols, and precipitation from EarthCARE: the ACM-CAP product, Atmos. Meas. Tech., 16, 3459–3486. https://doi.org/10.5194/amt-16-3459-2023
Wehr, T., T. Kubota, G. Tzeremes, K. Wallace, H. Nakatsuka, Y. Ohno et al., 2023: The EarthCARE mission – science and system overview, Atmos. Meas. Tech., 16, 3581–3608. https://doi.org/10.5194/amt-16-3581-2023