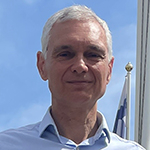
Massimo Bonavita, Principal Scientist, Data Assimilation Team Leader and Workshop joint organiser.
In May 2024, I was delighted, along with ESA colleagues, to welcome 173 scientists and engineers from diverse communities to ESA-ESRIN (Frascati, Italy) for the 4th edition of the ESA-ECMWF Workshop on Machine Learning for Earth System Observation and Prediction.
Representatives from satellite agencies, operational weather and climate prediction centres, industry and academia gave this workshop a truly diverse and multi-faceted perspective on the issues and highlighted the fact that ML technologies are increasingly transformative of current practices and assumptions.
In addition, we were joined by a large and active group of online participants (more than 600 registered attendees), which helped broaden the reach of the workshop to a global audience.
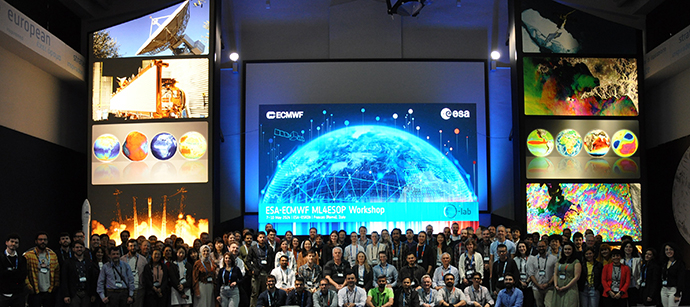
The 4th edition of the ECMWF-ESA Workshop on Machine Learning for Earth System Observation and Prediction attracted in-person and online participants totalling nearly 800.
Why so much interest?
In the first edition of the ML4ESOP workshop in 2020, the main focus was on how to integrate ML concepts and technologies into traditional numerical weather prediction (NWP) and climate prediction workflows to make them more efficient and effective. However, over successive workshops a clear trend has emerged in which researchers increasingly look to use ML to replace entire parts of current prediction systems, or even the entire NWP workflow!
While this development was expected, it is also undeniable that the pace of change has been supercharged by some of the big technology companies of our times entering the field.
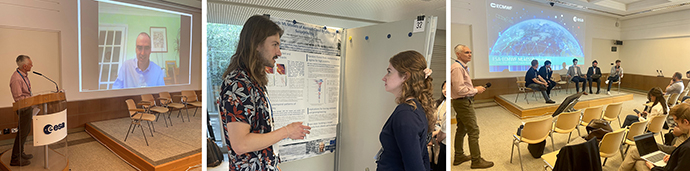
The workshop was a mixture of presentations, posters and working group discussions.
Where are we now?
ML for environmental monitoring
The use of ML techniques now seems ubiquitous in Earth observation, and new application areas are constantly emerging. For example, using ML technologies to enhance our observation and monitoring capabilities of emissions of greenhouse gases (GHG) and atmospheric pollutants is being explored by different research groups.
Monitoring of environmental conditions and related climate impacts (e.g. biomass, permafrost extent, polar sea ice, volcanic activity) with ML-augmented systems was also widely discussed, with results typically showing a positive impact with respect to more conventional approaches. The activities which ECMWF will lead in the second phase of Destination Earth (also presented at the workshop) will significantly boost the use of ML techniques for weather and climate applications. Exploiting the novel datasets of the DestinE digital twins, the main focus will be on developing workflows towards a machine-learning-based Earth system model. This will extend the work ECMWF is currently doing with the AIFS for the atmosphere, to components like ocean, sea ice or land, as well as towards longer (climate) timescales.
ML for weather and climate prediction
In the session on end-to-end ML for weather and climate, the latest advances in ML forecast models were presented, including ways to further improve their already impressive forecasting capabilities.
ECMWF’s Artificial Intelligence Forecasting System (AIFS) was shown to be competitive with the best performing ML models currently available, and a glimpse of the next generation of ML forecast models, based on generative AI techniques, was provided.
This next generation of ML models promises to overcome one of the main drawbacks of current ML models, namely their tendency to produce increasingly blurry and less sharp forecasts with increasing lead time, which was shown to be a fundamental limitation of training these models with mean squared/absolute error loss functions.
Another important limitation of the current generation of ML models is the fact they do not appear to respect basic physical conservation laws. In the working group discussion following the session, there was agreement that solving this problem will be a priority to increase confidence in and interpretability of ML-model forecasts.
Hybrid ML, NWP and climate prediction workflows
This session was mainly dedicated to research on embedding ML tools inside traditional NWP and climate workflows. Examples included efforts to use ML for the estimation and correction of the ECMWF IFS model error; preliminary work aimed at building a data-driven observation operator for surface-sensitive satellite radiances; and a demonstration in a toy model of how it would be possible to use generative AI techniques (e.g. diffusion models) inside a traditional data assimilation system.
Monitoring and forecasting the evolution of sea ice is a topical subject in the current changing climate. It is a particularly promising area for hybrid physics-based and data-driven methods because of the increasing amount of satellite observations available for monitoring sea ice combined with the inherent complexities and uncertainties of physics-based models of sea ice.
Hybrid ML, NWP and climate prediction at sub-seasonal to seasonal timescales
The sub-seasonal to seasonal forecast horizon used to be the domain of statistical forecasting methods until the advent of coupled dynamical models in the late 1990s. Recent ML developments seem to have turned the pendulum back towards data-driven prediction at these forecast ranges, but smart solutions combining dynamical and data-driven models can in fact surpass either of the two approaches in isolation.
An illuminating example was the combination of a dynamical model with an ML model to provide vastly improved predictions of the South Asian monsoon. This hybrid model presents realistic variability given by its physical component, but is also able to constrain the growth of errors on monthly timescales due to its ML component. The application of ML to the prediction of summer drought in Europe was another interesting example.
One of the big challenges for data-driven methods in seasonal prediction is the relatively limited training dataset (i.e. there are not enough seasons available in current reanalysis datasets), and the problem is made worse by the appreciable shift in the distribution due to ongoing climate changes. A way to tackle this problem is to use dynamical models for climate simulations and use these simulations to augment the training dataset.
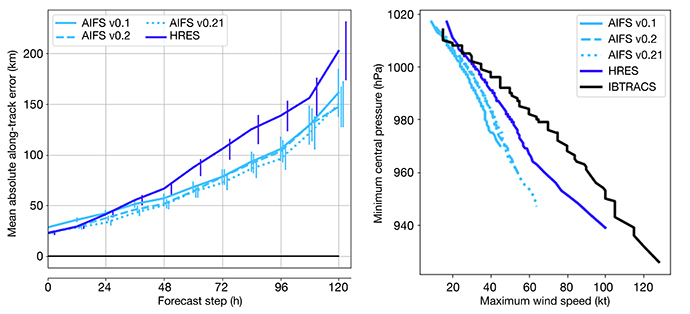
While ECMWF’s AIFS performs better in forecasting tropical cyclone (TC) tracks compared with the physics-based IFS (left), its physical representation of TCs is even further from reality (right). Left: mean absolute error of tropical cyclone track forecasts with the operational IFS model compared with successive versions of the AIFS ML model. Right: the relationship between minimum sea-level pressure and maximum wind speed for tropical cyclone forecasts with the IFS and AIFS compared with observations (IBTRACS). Data is for one year. Courtesy of Mihai Alexe, ECMWF.
Looking into the crystal ball
It is well known that making predictions is difficult and this is even more the case in ML, where the pace of progress is relentless! Conscious of the dangers, I will still offer a few personal thoughts on the possible evolution of some of the main areas discussed in the workshop.
After the initial rapid rise of ML forecast models trained on ECMWF reanalysis datasets, some important challenges are coming to the fore. Developing independent ML-based forecast systems would mean tackling the considerably more difficult problem of using observations directly and in a regular cycle of data assimilation, rather than relying on NWP-derived analyses and short-range forecasts.
On a more fundamental level, going from observations to predictions will require the ML community to effectively reinvent data assimilation (DA). This is certainly possible, but it is unclear that it will reach a significantly different endpoint to where we are now, considering that current DA techniques are based on a robust theoretical Bayesian framework.
The other challenge for ML models is to provide physically consistent forecasts. This is important to make the ML forecasts interpretable, which is crucial from a decision-maker point of view. There is no intrinsic guarantee that purely data-driven models respect physical conservation laws unless explicitly or implicitly constrained to do so during the training phase. The next phase of ML model development will likely see increased efforts in this direction.
We live in a Big Data era, where a proliferation of Earth observation sensors provides us with ever-increasing coverage. On the other hand, it is unlikely that even this huge amount of information will ever completely observe the Earth system. Developing good knowledge-based models will still be crucial to fill in the gaps between observations, to extrapolate to unobserved quantities and, in general, to build a coherent picture of the world we live in.
So, in my view, the most likely outcome of the current ML revolution in the Earth sciences will be the emergence of more efficient hybrid models that skilfully combine knowledge-based and data-driven models. For this programme to be realised, a close collaboration between ML and domain scientists will be crucial.
Next steps
Presentations and recordings of the workshop will be made available on the main workshop website.
As in previous editions, a peer-reviewed paper discussing the main outcomes of the workshop will be published in Nature npj Climate and Atmospheric Science.
The next edition of the workshop will be hosted by ECMWF and is provisionally scheduled for the first half of 2026.
For more information, read ESA's article about the workshop.