
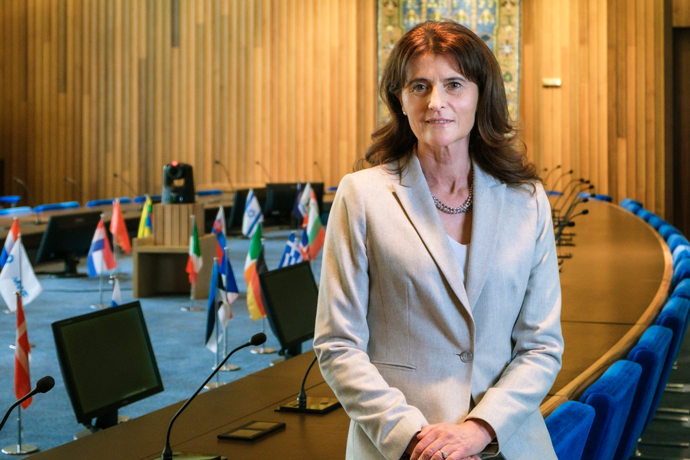
Machine learning is set to play a growing role in numerical weather prediction, but physics-based forecasting techniques will continue to be important, ECMWF’s Director-General Florence Rabier has said in an interview. She explained that ECMWF is currently pursuing a three-pronged approach: the use of machine learning to boost traditional techniques, the development of a pure machine-learning forecasting model, and an experimental use of machine learning on weather observations to build a forecasting system. For the longer term, we will start investigating the design of a foundation model for weather and climate.
Machine learning has entered the field of numerical weather prediction in recent years. Why does machine learning matter to ECMWF?
Artificial intelligence and machine learning (AI/ML) applications have recently expanded dramatically in all fields. ML uses a lot of data to produce results, and ECMWF is all about data: we use a lot of data – tens of millions of weather observations per day – and we create a lot of data by producing weather analyses and forecasts at high resolution.
Traditionally, we use physics-based methods for our computations in the Integrated Forecasting System (IFS). This is the case for numerical weather prediction (NWP), reanalyses of past weather, and atmospheric composition. All computations require large computing power to produce high-quality predictions, based on the accurate description of physical processes.
ML can enhance our computations by adding components or replacing some parts of the process. This is what we call the ‘hybrid’ approach. Instead, ML can also fully replace the whole model, at a much lower computing cost. In addition, AI/ML opens some avenues in other activities we are involved in, such as flood forecasts, fire danger forecasts, monitoring of observations, managing the supercomputer, or enhancing user experience.
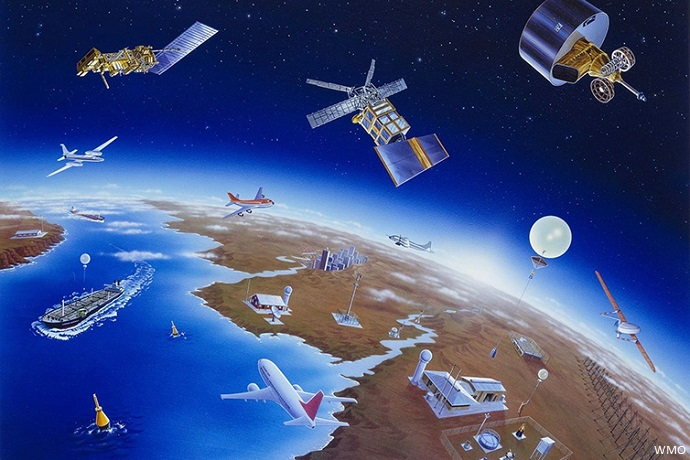
Machine learning is well suited to weather forecasting due to the vast amounts of data that are used to determine the best possible initial conditions and to produce global forecasts.
How does the hybrid approach work?
Machine learning can be used to better estimate some components of NWP, and to estimate model error. This is true for the process of establishing the best possible starting conditions of forecasts, called data assimilation, but also for forecasts themselves.
Aspects of the Earth system, such as sea ice, snow, soil and vegetation, are hard to model from pure physics, so in practice, the modelling can be quite empirical. In such cases, there is great potential to let the observations increasingly define the models. As we have demonstrated for sea ice assimilation, we are more likely to achieve the best results by carefully combining known physics with empirical and ML components, rather than throwing away physical models entirely.
There are also some predictable model errors which we can estimate and remove from data assimilation and subsequent forecasts using ML. Weather and climate reanalyses can benefit from ML, too. For example, for ECMWF’s next reanalysis, ERA6, an ML model error correction method developed for the period from 2006, which is rich in satellite observations, will be applied to earlier, data-sparse periods.
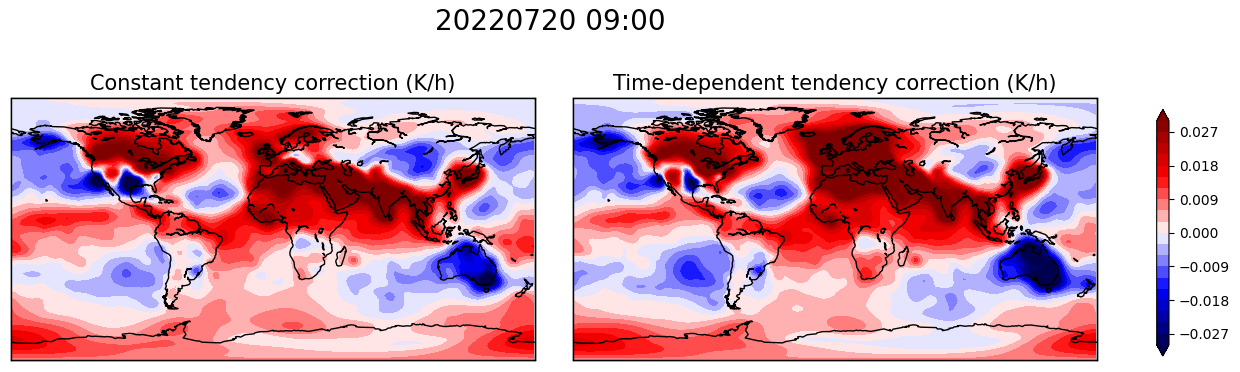
This animation illustrates the variable corrections of near-surface temperature tendencies per hour, established by machine learning, that are going to be applied in the data assimilation system from 2025, compared to constant tendency corrections. The constant tendency correction includes small changes for every 12-hour assimilation window. (Time series courtesy of Patrick Laloyaux, ECMWF)
What kind of progress has been made on a forecasting system based purely on ML?
We have made tremendous progress in the last 18 months or so in building such a system, called the Artificial Intelligence Forecasting System (AIFS). This is the result of decisions by our Member States on investments, the motivation and dedication of our staff, and collaborative efforts with partners. The AIFS uses Copernicus climate reanalyses and weather analysis for training, and it relies on IFS initial conditions, so it is not entirely divorced from traditional techniques. But it makes forecasts just on the basis of ML.
This year we have lowered the grid spacing of the AIFS from 100 to 28 km, and it will go down further. This compares with a grid spacing of currently 9 km for the IFS. We have also built a first AIFS ensemble system, in which a number of slightly different forecasts are made for each time in the future to scan the possible scenarios and thus enable the estimation of uncertainty. These forecasts have been added to our charts web page, and they are available as open data.
We are looking at sub-seasonal timescales, up to 46 days ahead, and at the addition of various Earth system components. There are also plans for AIFS forecasts of hydrology and atmospheric composition. We are collaborating in an ECMWF ML pilot project with Member and Co-operating States, and we are taking part in a EUMETNET AI initiative. This will help us to compare different approaches, at different timescales and resolutions. The collaboration with our Member and Co-operating States is also key to the development of a platform called Anemoi, which will enable users to build their own ML models.
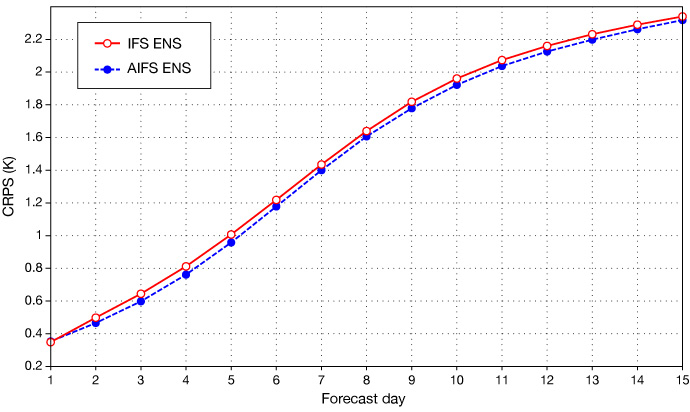
The continuous ranked probability score (CRPS – lower is better) measures the quality of ensemble forecasts. Here we show it for 850 hPa temperature, with results for the IFS ensemble (IFS ENS – red) and for the experimental AIFS ensemble (AIFS ENS – blue). Scores are aggregated over the northern hemisphere extratropics and approximately a five-month period.
What is the status of using ML just on observations?
Over the last year, we have started a radically new approach to weather prediction: the production of weather forecasts directly from observations. Physics-based data assimilation relies on some assumptions, such as a perfect knowledge of model and observation errors, and of the link between the model and observations. The new approach tries to circumvent these aspects of conventional data assimilation.
It means that observations do not need to be mapped to a fine grid of unmeasured parameters. It also opens up the possibility of exploiting the information content of exciting new observations. This AI-Direct Observation Prediction (AI-DOP) project involves a neural network trained to predict future observations from long historical records of past observations.
In this new approach, we effectively use observations to forecast future states of the atmosphere learned directly from the observations themselves. First results are very promising, but this approach is still experimental.
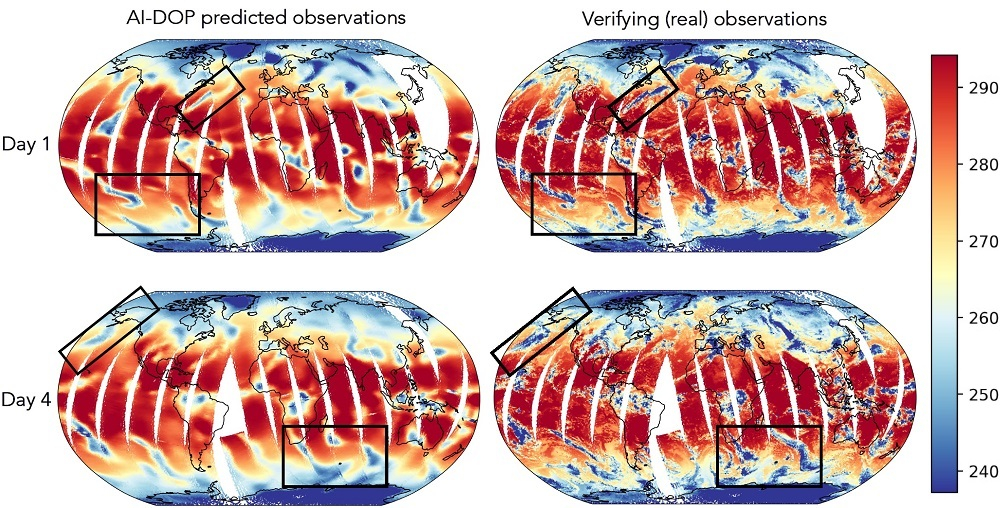
An example of forecasting future observations with AI-DOP. We show infrared window channel brightness temperatures (in Kelvin), measured by the Infrared Atmospheric Sounding Interferometer (IASI), predicted one and four days ahead. The left column shows the predicted values and the right column real radiances measured one and four days later. This channel is very sensitive to atmospheric cloud structures, and we can see that AI-DOP produces very plausible forecasts of the evolution of the large-scale weather patterns, although they are rather smoothed with the current low-resolution experimental system. The rectangles highlight some of the more conspicuous weather patterns that are predicted well.
What does the future hold?
In 2025, the AIFS ensemble system will be made operational as a complement to the IFS. Work will continue to further improve ML modelling and increase the spatial resolution, moving towards 9 km. Beyond the medium range, both hybrid and data-driven approaches will be developed for sub-seasonal and seasonal forecasting systems. An operational AIFS for these timescales will be developed by 2026.
Within the EU’s Destination Earth initiative, the scope of the AIFS will be expanded to capture ocean, sea ice, land, hydrology and wave processes. ECMWF could also contribute with its broad expertise and resources to support the AI Factories of the European Commission. Within the EU’s Copernicus services, a hybrid and an AIFS atmospheric composition model will be explored, as well as machine-learning-based methods for downscaling the ERA5 global reanalysis to European and Arctic regions. The direct use of observations in ML model development will continue.
In collaboration with partners across the European meteorological community, we are also planning to develop a foundation model for weather and climate by 2027, using a large variety of data and having the possibility to be adapted to a wide range of tasks. For example, it will function as a forecasting, downscaling and post-processing tool. It will apply across sectors such as weather, water, energy, health and food security.
Will there always be a role for a physics-based forecasting system?
ECMWF strives to be at the forefront of AI/ML developments, together with the European Meteorological Infrastructure, to support the continuing goal of world-leading weather forecasting. That does not mean that we are giving up on physics-based forecasting. Much current work is about how best to combine the two to produce the best possible results.
We are, for example, planning to develop higher-resolution small ensembles of the physics-based model to complement the current production at a grid spacing of 9 km. This will be helpful in its own right, but it will also help to train data-driven models, which will also go to higher resolution.
From what I have described, you can see that in the future there will still be a role for physics-based modelling. It will serve to anchor the system, even if an increasing part of operational production is performed with data-driven approaches.