
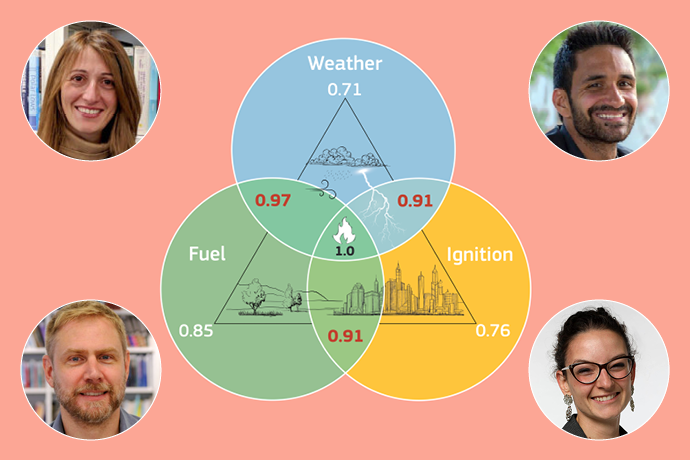
Francesca Di Giuseppe, Joe McNorton, Anna Lombardi and Fredrik Wetterhall (clockwise from top left) are the authors of the paper published in Nature Communications.
Our scientists have developed a new tool for improved wildfire prediction using machine learning (ML), as set out in a paper published on 1 April 2025 in Nature Communications.
The paper describes how the collection and integration of higher-quality data can significantly improve the accuracy and reliability of wildfire predictions.
It evaluates how our new data-driven fire danger forecasting model, the Probability of Fire (PoF), performed in 2023 and in recent extreme events.
Our role in fire prediction
We have been producing fire danger forecasts since 2018 as part of the Copernicus Emergency Management Service (CEMS) led by the European Commission’s Joint Research Centre.
In recent years, we have developed innovative approaches using ML methods. This has enabled us to move from predicting fire danger – a measure of landscape flammability – to forecasting fire activity.
The new products, using the Probability of Fire model, are distributed to CEMS and are accessible to ECMWF Member States.
The new model
The new Probability of Fire model incorporates multiple data sources beyond weather prediction to identify areas at risk of ignition.
“Thanks to a machine learning algorithm, it takes a more holistic approach,” says the lead author of the Nature Communications article, Francesca Di Giuseppe. “Traditional weather-based fire danger indices often fail to pinpoint areas at risk of ignition with enough specificity. This is where ML can help.”
For example, in the case of the Los Angeles fires in January 2025, weather forecasts identified broad areas as very flammable but did not accurately target the most probable ignition hotspots.
By incorporating additional parameters, such as human presence, lightning activity and, most importantly, vegetation abundance and its dryness, a more accurate and targeted fire risk can be established. Including all data sources improves the model’s predictive skill by up to 30%.
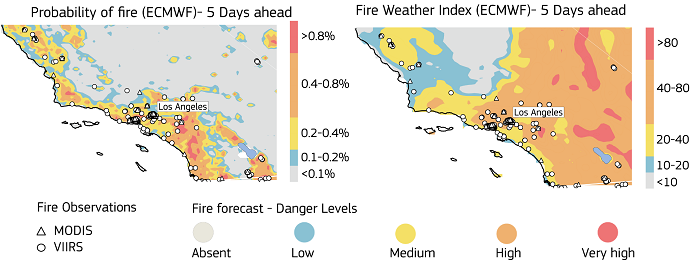
Southern California 1-day forecast of fire danger from the Probability of Fire model (left) and the traditional Fire Weather Index, which is the headline metric used in the GWIS and many other early warning systems regionally (right) for 7 January 2025. Active fire observations from MODIS (triangles) and VIIRS (circles) for the same day are overlaid.
Fuel information is unlike weather data in that it is not easily obtained through direct observations or prediction systems. However, ECMWF has used its weather forecasting expertise and data from the EU’s Copernicus Atmosphere Monitoring Service (CAMS), which is implemented by ECMWF, to construct a modelling framework to extract this information.
"In this research, we found that high-quality data, such as information on vegetation moisture and fuel availability, is the most important factor for improving forecast accuracy,” says Joe McNorton, another ECMWF contributor to the study. “The Probability of Fire model's ability to capture these changes demonstrates how ML-driven models are increasingly crucial for accurate wildfire predictions and emergency preparedness.”
Further resources
In addition to the Nature Communications paper, more details on the Probability of Fire model are contained in a recent ECMWF blog post and in a video explainer.