
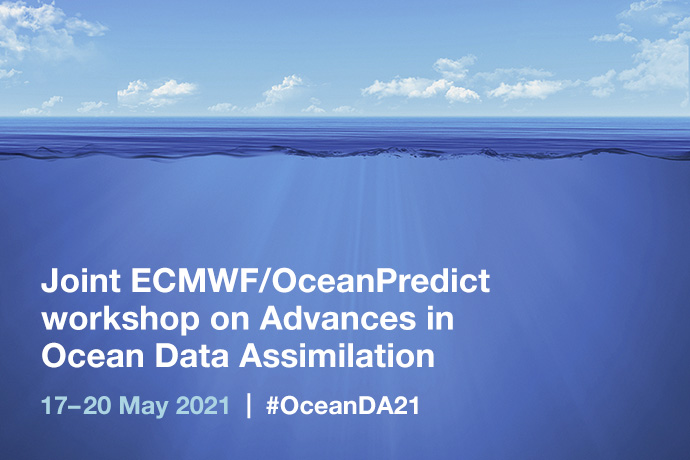
Some 170 scientists from around the world presented recent progress and challenges ahead in ocean data assimilation in a virtual event from 17 to 20 May 2021.
During this workshop, organised jointly by ECMWF and OceanPredict, experts addressed the science underpinning climate and environmental monitoring and predictions, the exploitation of novel observations, and interactions in the ocean–atmosphere–sea-ice–biochemistry system at global and regional scales.
The event was designed to meet the ever-increasing requirements of marine, weather, environmental and climate services. It was very timely since 2021 marks the beginning of the U.N. Decade of Ocean Science for Sustainable Development.
Bringing together communities from different disciplines
The workshop provided a unique opportunity to address multi-disciplinary topics by bringing together experts from different domains.
It addressed what is common to all applications, such as the treatment of model error, the specification of short-range forecast and observation errors, and the exploitation of machine learning.
It also looked into infrastructure needs to share developments among different domains, and between operations and research.
"One of the best aspects of the workshop was the interaction of data assimilation scientists from diverse application areas,” says Massimo Bonavita, who leads the data assimilation team at ECMWF. “While general methodologies are very similar, the realisations of the different constraints in different Earth system domains provides people with new perspectives and helps generate new ideas."
A virtual but vibrant hub
A combination of plenary talks, poster sessions, working group discussions and informal virtual breaks facilitated the exchange of information and seeding of new ideas.
The event included several virtual meeting places, including ECMWF grounds.
“I really enjoyed the workshop. It was great to see a wider diversity of talks enabled by the online platform,” says Sergey Frolov, a data assimilation expert from the NOAA Physical Science Laboratory. “I was at first sceptical about the ability of the workshop to capture the informal interactions that we expect of the in-person workshop. However, the gather-town platform captured some of this magic."
Progress and future directions
In the past few years, operational ocean forecasting activities have consolidated in different centres, and the number of ocean specialists has increased. That means there is now a critical mass to spark good collaborations.
“The increasing number of ocean applications implies that the community is getting more diverse, which also contributes to progress in the field,” points out Yosuke Fujii from JMA. “Oceanic ensemble generation and the detailed design of the background error statistics have made impressive progress in the last two or three years.”
This impression is confirmed by Anthony Weaver from CERFACS in France: "Methods used for operational ocean data assimilation have traditionally been somewhat less sophisticated compared to those used for numerical weather prediction. Work presented at the workshop indicates that the gap has narrowed significantly, notably with the development of ensemble-variational methods for ocean data assimilation at ECMWF and other operational centres.”
Progress is being made in the new field of coupled data assimilation, where both technological and scientific developments were presented at the workshop.
The working group discussions acknowledged the complex science of coupled data assimilation. They recommended the training of experts in the ocean–atmosphere boundary layer and machine learning, and the use of targeted observations of the interface for process understanding and modelling improvements.
Small group discussions were part of the programme.
“While many challenges still remain, particularly in relation to coupled data assimilation, it was great to see all of the recent progress that has been made in support of ocean and climate science, and OceanPredict is very excited about the new intersection of data assimilation with machine learning," says Andy Moore, co-chair of the OceanPredict task team on data assimilation.
Oral and poster sessions presented progress on variational and ensemble methodology and algorithms, theoretical considerations on the validity of methods for different degrees of non-linearity, and practical considerations on balancing resolution and the number of ensemble members.
They also considered methods for quantifying observation impact, assimilating novel observations, and better exploiting the existing observing system, as well as algorithms for improved ensemble generation and computational efficiency.
Directions for progress were further discussed in the working groups.
Research posters could be discussed online.
Infrastructure and expertise
Beyond methodology, the workshop also touched on common developments for sharing data assimilation infrastructure, with major initiatives such as JEDI and PDAF being discussed.
The need for data-sharing infrastructure to facilitate collaborations was also identified. This is especially relevant for sharing expensive experiments that only a few operational agencies are able to conduct.
A clear and important recommendation from the workshop was the need to strengthen the data assimilation research in non-operational centres, and to prepare the next generation of data assimilation scientists.
To this end, sustained research funding for data assimilation in non-operational centres is required to create a sufficient pool of expertise to exploit new computer architectures and observing systems.
More information
For more information, including all posters and recordings of the talks, please visit the workshop page on the ECMWF website.