
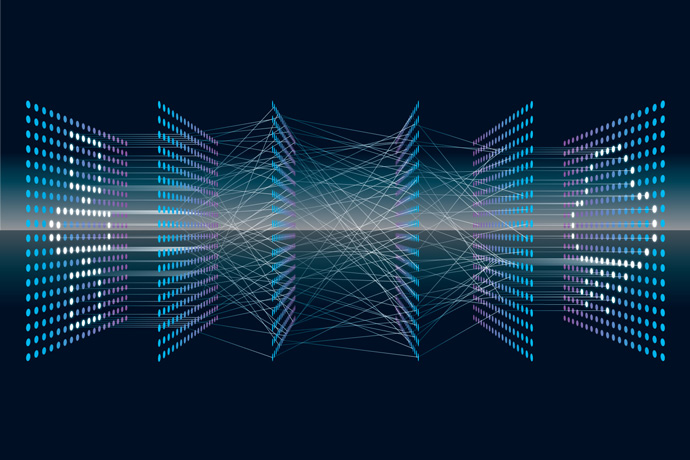
ECMWF is organising a series of seminars given by international experts to explore aspects of the use of machine learning in weather prediction and climate studies.
The first will take place on 28 April and will be live-streamed. Sherman Lo and Ritabrata Dutta from the University of Warwick will present a statistical methodology to predict precipitation at 0.1° resolution using lower-resolution model fields of air temperature, geopotential, specific humidity, total column water vapour and wind velocity.
On 9 June, Annalisa Bracco from the School of Earth and Atmospheric Sciences at the Georgia Institute of Technology will talk about spatiotemporal complexity and time-dependent networks in mid- to late Holocene simulations.
In subsequent seminars, Maxime Taillardat (Météo-France) will present examples of operational ensemble post-processing using machine learning; Alberto Arribas (UK Met Office) will talk about work at the Met Office Informatics Lab; and Nal Kalchbrenner (Google) will talk about now-casting applications at Google. More seminars will follow later in the year.
For up-to-date details and joining instructions, visit the ECMWF web page on the seminar series.
Machine learning at ECMWF
“ECMWF is currently making a significant effort to identify how artificial intelligence and machine learning applications can improve numerical weather prediction,” says Peter Düben, ECMWF’s coordinator for artificial intelligence and machine learning activities.
In January 2020, an internal machine learning workshop revealed that there are some 25 projects at ECMWF that are using or are going to use machine learning. Applications are spread over the entire numerical weather prediction workflow. Examples include:
- bias correction of satellite observations
- the learning of model error within data assimilation
- the emulation of model components to increase computational efficiency in the forecast model
- local downscaling of model output to improve predictions
- the monitoring of the IT infrastructure.
Potential areas of application for machine learning are spread over the entire numerical weather prediction workflow.
“In the future, the European Weather Cloud, which is being developed jointly by ECMWF and EUMETSAT, is likely to play a very significant role in the development of machine learning tools,” Peter says.
He points out that researchers with access to Cloud computing resources will be able to easily load training data from the data archive and to use standard machine learning software tools such as TensorFlow and Jupyter notebooks.
In addition to the seminar series, ECMWF and the European Space Agency (ESA) are organising a joint workshop on ‘Machine Learning for Earth System Observation and Prediction’ that will take place at ECMWF from 5 to 8 October 2020.
“Machine learning is definitely growing in importance in numerical weather prediction,” Peter says. “The seminar series and the workshop will provide new perspectives to inform future work in this rapidly developing area.”