
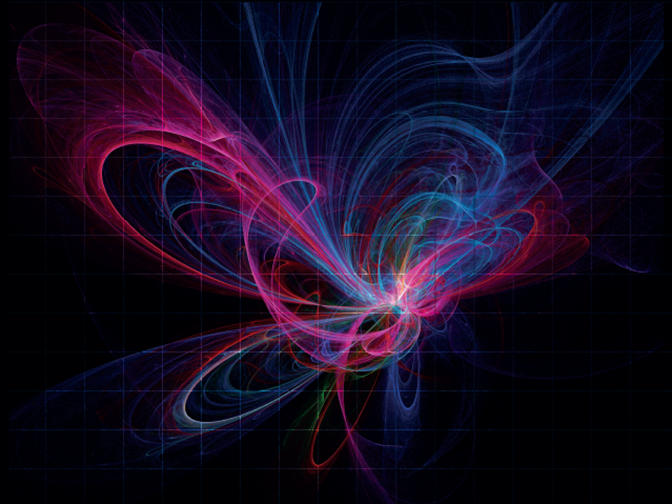
Seventy-eight scientists from 15 countries met at the Centre from 11 to 15 April to discuss how best to deal with uncertainties in the models used in weather and climate forecasting systems.
The complexity of the Earth system and limitations in computing power mean that some of the processes involved in weather can only be modelled approximately.
The key question of the workshop was how to make reliable forecasts in the face of such approximations.
The production of multiple forecasts, known as ensemble forecasting, allows model and initial uncertainty to be accounted for in the forecast. Increasing the reliability of forecasts therefore hinges on improving the representation of model uncertainty in the ensemble.
Scope for improvement
ECMWF Director of Research Erland Källén noted that, while there has been steady progress in the skill of ensemble forecasts, there is room for further improvement.
“It is reassuring to know that we are making progress, but some forecasts are worse than others,” he said on the first day of the Workshop on Model Uncertainty, organised jointly by the Centre and the World Weather Research Programme.
“We have to understand where the uncertainties come from, both model uncertainties and uncertainties in the state of the atmosphere used to initialise a forecast,” Professor Källén said.
Paolo Ruti, the chief of the WMO’s World Weather Research Division, highlighted the challenges an Earth system approach brings.
“Increasingly we are working on coupled systems, so model uncertainty is no longer just a problem related to the atmosphere,” he pointed out.
ECMWF Lead Scientist Roberto Buizza observed that model uncertainties are one of the sources of forecast error and “should thus be simulated in ensemble systems”. “We want to make ensembles more reliable and more accurate,” he said.
Collaborative approach
Thirty speakers presented their research on model uncertainties, and participants discussed the way forward in working groups dedicated to three questions:
- What are the sources of model uncertainty and how do we improve the physical basis of model uncertainty representation?
- How can we improve the diagnosis of model error?
- What are and how do we measure the pros and cons of existing approaches?
Sarah-Jane Lock, a scientist in ECMWF’s Model Uncertainty Group and one of the workshop organisers, said the discussions had been stimulating and would feed into future research.
“The workshop confirmed that the topic of model uncertainty is inherently difficult and that getting the international community together is essential to identify the best ways forward,” Dr Lock observed.
Many of the scientists presented posters summarising their research.
In order to identify model error, we need some measure of the ‘truth’, and this is not as straightforward as it may seem.
The truth will never be fully observed due to limitations in the available observations. Moreover, the finite resolution of forecasting systems means that a single model state could represent multiple different real states.
“There was broad agreement that recent progress in data assimilation offers promising methods to identify and represent model error,” Dr Lock noted.
“However, greater efforts are required to relate the work carried out in the data assimilation community to that done by forecast model developers, and vice versa.”
The way ahead
Recommendations from the working groups include continuing the effort to build model uncertainty representations that are closely tied to uncertain processes; to improve our understanding of the upscale growth of errors in our models; and to better exploit observations for process-based verification.
It was emphasized that model errors derive not only from the unresolved scales but also from scales well resolved by the models. Consequently, seemingly broad-brush uncertainty representations may continue to be required.
“The workshop highlighted that there are many new and emerging approaches to explore for diagnosing model error as well as deriving new model uncertainty representations and verifying their impact,” Dr Lock said.
“Representing model uncertainty is an integral component of any ensemble numerical weather prediction system, and this topic will continue to be a very active area of research in the coming years.”
Nearly 80 scientists from 15 countries took part in the Model Uncertainty Workshop.