← prev Ensemble forecasting for anticipatory humanitarian action |
Contents |
next → Side-stepping ‘futility’: Tim Palmer on energy-momentum in general relativity |
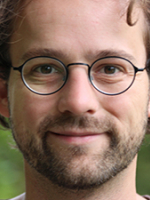
Peter Düben
ECMWF
Today, ensemble forecasts are at the heart of weather prediction at ECMWF and work on ensembles is performed across the workflow of the numerical weather prediction production chain. An ensemble of initial conditions is generated via ensemble data assimilation. The initial conditions are then adjusted via the use of singular vector perturbations and used to generate 50-member medium range, extended range and seasonal prediction ensembles. However, ensembles are also used in hindcasts and for ERA5 reanalysis and they also play a big role in the forecast products of ECMWF, such as the heavy used ensemble Meteograms.
The ensemble prediction system is constantly upgraded via new model cycles of the Integrated Forecast System (IFS). One of the larger changes during recent years was the reduction of numerical precision in the representation of model variables from double precision (with 64 bits per variable) to single precision (with 32 bits per variable) in the atmosphere, land and wave model which is reducing compute time for high-resolution forecast simulations by around 40%. The reduction in precision in the IFS was explored first by Tim Palmer’s group in Oxford with the OpenIFS model and was justified by Tim due to the inherent uncertainty in all forecast simulations due to imperfect initial conditions and the truncation of sub-grid-scale information in the discrete models. This justifies a trade between precision and performance in forecast simulations of chaotic systems. In IFS model cycle 47R2, the savings from a precision reduction could be reinvested into an increase of vertical resolution from 91 to 137 vertical levels which allowed for good improvements in forecast scores while reducing the actual computational cost of forecast simulations (Lang et al. 2021).
In the next IFS model cycle 48R1, which will go operational in 2023, the resolution of the ensemble will be increased from 18km (TCo639) to 9km (TCo1279) grid-spacing and – for the first time – catch up with the resolution of the deterministic “high-resolution” forecasts at ECMWF. The following cycle 49R1 will switch from the Stochastically Perturbed Parametrization Tendencies (SPPT) to the Stochastically Perturbed Parametrization (SPP) scheme at all forecast lead times. Furthermore, ECMWF will introduce a new NEMO model (version 4) which will allow for the use of single precision for simulations of the ocean.
In the next four years, ECMWF will continue to extend SPP to represent uncertainties in surface processes of land, snow and ocean waves and improve the scheme at all lead times, for example via modifications of the probability distributions of the perturbed parameters. The stochastically perturbed semi-Lagrangian departure point (STOCHDP) scheme will be developed further to represent model uncertainties not only in the physical parametrization schemes but also in the dynamical core. Furthermore, it will be explored how to get the best results for uncertainty representation when adding simulations at km-scale resolution into the mix of forecast products. For km-scale simulations, variability will increase at small scales. While this would suggest keeping the number of ensemble members high, large number of ensemble members will be prohibitive due to the large computational cost. Furthermore, it remains to be seen how forecast quality will differ as resolution is increased from 4 to 1.5km – the maximal resolution that can currently be run (Wedi et al. 2020) – as we start to reach the end of the grey zone and an appropriate explicit representation of deep convection. As part of the DestinE project, ECMWF will study the impact of SPP on km-scale simulations and how km-scale and coarser resolution model simulations can be combined in the optimal way.
For the longer-term future, there may be new ways to trade between precision and performance, for example via the use of half precision (16 bits per variable) for specific kernels of the forecast models (Kloewer et al. 2022), or the emulation of parametrization schemes with neural networks which allow to perform similar trades as a more complex deep learning emulator will reduce the cost savings in comparison to conventional model parts, but also show smaller errors (Chantry et al. 2021).
On the use of machine learning, the representation of uncertainties will also be important for approaches of “hard-AI” (Chantry et al. 2020) which try to replace entire forecast models with machine learned models which are typically based on deep learning and trained from ERA5 reanalysis data (Rasp et al. 2020). These approaches could recently reduce root mean square errors (RMSE) significantly to a similar level of quality when compared to operational weather forecast models for specific model variables. The work is partly driven by big companies such as NVIDIA (Pathak et al. 2022). However, the machine learned forecasts are prone to smear out small scale structures for long lead times as they are typically optimised for a low RMSE – the next generation of these models will consequently need to focus on ensemble predictions and probabilistic measures of quality.
Even in the long-term future – which may see a change of the way we perform computing in the post-Moore era and maybe even a switch to other computer hardware such as quantum computers – the representation of uncertainties at all lead time will still be essential for our understanding of predictability and the usefulness of forecasts. In particular, as high-performance computers become more and more prone to hardware errors (Benacchio et al. 2022) and as quantum computers are noisy with interesting implications how we may represent uncertainty on such hardware. Luckily, someone has already started to think about how quantum computers could be used for probabilistic forecasts (Tennie and Palmer 2022).
← prev Ensemble forecasting for anticipatory humanitarian action |
↑ top | next → Side-stepping ‘futility’: Tim Palmer on energy-momentum in general relativity |