← prev Projectability |
Contents |
next → The benefits of a seamless approach to prediction |
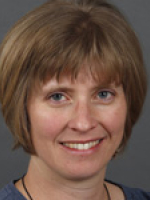
Judith Berner
NCAR, USA
As we heard in a toast by Martin Miller at the “ECMWF Symposium on Ensemble Forecasting for Prof. Tim Palmer” in Reading on December 5, 2022, some key scientific ideas are generated in airport launches due to delayed flights. According to this toast this was how the idea for the ground-breaking paper on “Stochastic representation of model uncertainties in the ECMWF ensemble prediction system” by Tim Palmer and his colleagues Roberto Buizza and Martin Miller was conceived (Buizza et al., 1999).
In the 1990s, the atmospheric sciences embraced stochastic methods. Penland and Sardeshmukh published their work on linear inverse modeling and how interactions between non-normal modes can result in temporary energy growth (Penland and Sardeshmukh, 1995).
Work by Frank Selten showed that the 3rd EOF of the Lorenz 63 system can be replaced with a stochastic process without the loss of its characteristic regime behavior (Palmer, 2001). This is an example how stochastic processes can increase the dimensionality of a dynamical system and counteract the shortcomings of truncation.
Subsequent work by Tim and colleagues showed that stochastic parameterizations work best when non-locality via spatial and temporal correlations is introduced (Palmer, 2001). This can be done via cellular automata (e.g. Shutts, 2005) or a spectral pattern generator (Berner et al., 2009). Such spatial and temporal correlations are motivated by the aim to represent mesoscale organization in coarse-resolution models.
In the following decade various stochastic schemes were implemented at a number of operational and academic centers. The most popular schemes were the “stochastically perturbed physics tendency scheme” (SPPT, Palmer et al., 2009), the stochastic kinetic-energy backscatter algorithm” (SKEBS, Shutts et al., 2005) and the “stochastically perturbed parameter scheme” (SPP, Ollinaho et al., 2017).
Besides improving forecast skill by increasing reliability, stochastic schemes have shown to improve blocking (Jung et al., 2005), systematic errors in climate (Weisheimer et al., 2014, Berner et al., 2017) and climate sensitivity (Strommen et al., 2019).
As we are entering the era of global cloud-permitting weather models, the field of stochastic parameterizations is evolving but will likely stay as a way to represent unresolved and unrepresented subgrid-scale uncertainty.
← prev Projectability |
↑ top | next → The benefits of a seamless approach to prediction |