← prev How ensemble forecasts enhance the energy sector |
Contents |
next → Some thoughts on the contributions of Tim Palmer |
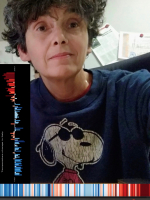
Susanna Corti
ISAC-CNR, Italy
Thirty years ago, the 24th of November 1992, ensemble forecasting became operational at ECMWF. Thirty years ago, I was a PhD student at the Physics Department of the University of Bologna and in the spring of 1992, I visited (for the first time) ECMWF to attend the Training Course on Numerical Weather Prediction. Tim used to give the introductory lecture for the last module: Predictability. This was “Introduction to Chaos”, and, finally, I felt at home. The previous couple of weeks during the lectures on Data Assimilation, Numerical Methods and Parametrizations of sub-grid Physical processes I had gotten slightly lost. My background in Physics, with various insights into Theoretical Physics and Dynamical Systems, had not been of much use. But at the end of Tim’s lecture, I realised that my previous studies might have practical applications after all, and this was a very exciting discovery. And of course, research conducted by Tim and the Predictability section of the ECMWF Research Department became my main reference. Less than one year later (in February 1993) I joined ECMWF for 8 months as a visiting PhD Student and I started “practicing research” in the Predictability Section. After, my PhD my collaboration with Tim continued at constant (yet irregular) intervals, both at ECMWF and remotely. I must say that for me it has been an extraordinary (and enlightening) scientific adventure.
Following the famous definition by Edward Lorenz, weather and climate predictability problems can be divided into two main categories. In the first category (No.1) fall predictions of the evolution of a system given some knowledge of its initial state, and predictability is primarily an initial-value problem. Because the atmosphere is chaotic, predictability is associated with the local instabilities of the flow: it varies around the (unknown) atmospheric attractor (flow dependent predictability). If we knew the structure of the atmospheric attractor and its error growth properties as we know those of the Lorenz63 model, we could forecast the forecast skill. In other words, we could give an (a priori) estimate of confidence in a forecast. On the other hand, predictions aiming to estimate how (the attractor of) a given dynamical system responds to a change in some prescribed parameter or external forcing belong to Lorenz’s second category (No.2). In this second case, uncertainties may arise from the accuracy in prescribing the forcings themselves and the accuracy in model response to changes in the forcings. Quite obviously a numerical weather forecast (as a forecast of El Niño) belongs to No.1. By contrast, estimating the effects on climate of prescribed anthropogenic changes in atmospheric composition (or land use) would naturally fall in No.2. This Lorenz pragmatic “cut” is a very useful concept, but, in practice, many forecasts do not fall exclusively into one of these two categories to the exclusion of the other one. In other words, the intersection between set No.1 and set No.2 is not the Empty set. Let’s call it set No.1/2. It contains all those forecasts for which predictability arises from both components: the correct initialisation and changes in external forcings.
In the research I carried out, supervised and in collaboration with Tim, I had the privilege to explore all these two and a half aspects of predictability, both in (relatively) simple and complex models. We studied flow dependent predictability in a QG model and we identified fairly distinct paths in phase space associated with predictable and unpredictable transitions, qualitatively similar to that found in the Lorenz63 model. We investigated how predictability arising from changes in the boundary conditions and external forcing might be intimately linked to the (correct) simulations of natural circulation regimes and their associated variability. We showed that the simulation of such regimes improves considerably when increasing model resolution. At last, we focussed on near-term climate predictions, the hybrid problem whose elements populate set No. 1/2. Near-term climate predictions from one to several years in advance represent the natural extension of seasonal predictions, so they can be considered as initial-value problems where the correct initialization of the ocean together with the sea ice plays a central role in keeping the trajectory of the system close to the observed one. However, as lead time increases from one season to several years, the component of predictability arising from changes in the external forcing becomes more and more important. In this context we assessed (for the first time) the reliability of multi-year predictions using probabilistic Attributes Diagrams for near-surface air temperature and sea surface temperature. We found that over the North Atlantic the hindcasts are both sharp and reliable, even after detrending, confirming the importance of initialisation in that region. To study the relative impact of initialisation versus external forcing we used specifically designed sensitivity experiments consisting in two sets of 10-year-long ensemble hindcasts for two initial dates, in which external forcing and initial conditions were swapped. By comparing the two sets of integrations, the impact of external forcing versus initial conditions on the predictability over multiannual time scales was estimated as the function of lead time of the hindcast. We found that the correct initialization has a multi-year impact on SST predictability over specific regions such as the North Atlantic, the north-western Pacific, and the Southern Ocean. The impact of initialization is even longer and extends to wider regions when below-surface ocean variables are considered, extending to as much as 9 years in some regions.
Well, that’s that ... Yet, I hope that there will be more opportunities in the future to collaborate with Tim. For now: Happy Birthday Ensemble Forecasting! Happy Birthday Tim Palmer!
← prev How ensemble forecasts enhance the energy sector |
↑ top | next → Some thoughts on the contributions of Tim Palmer |